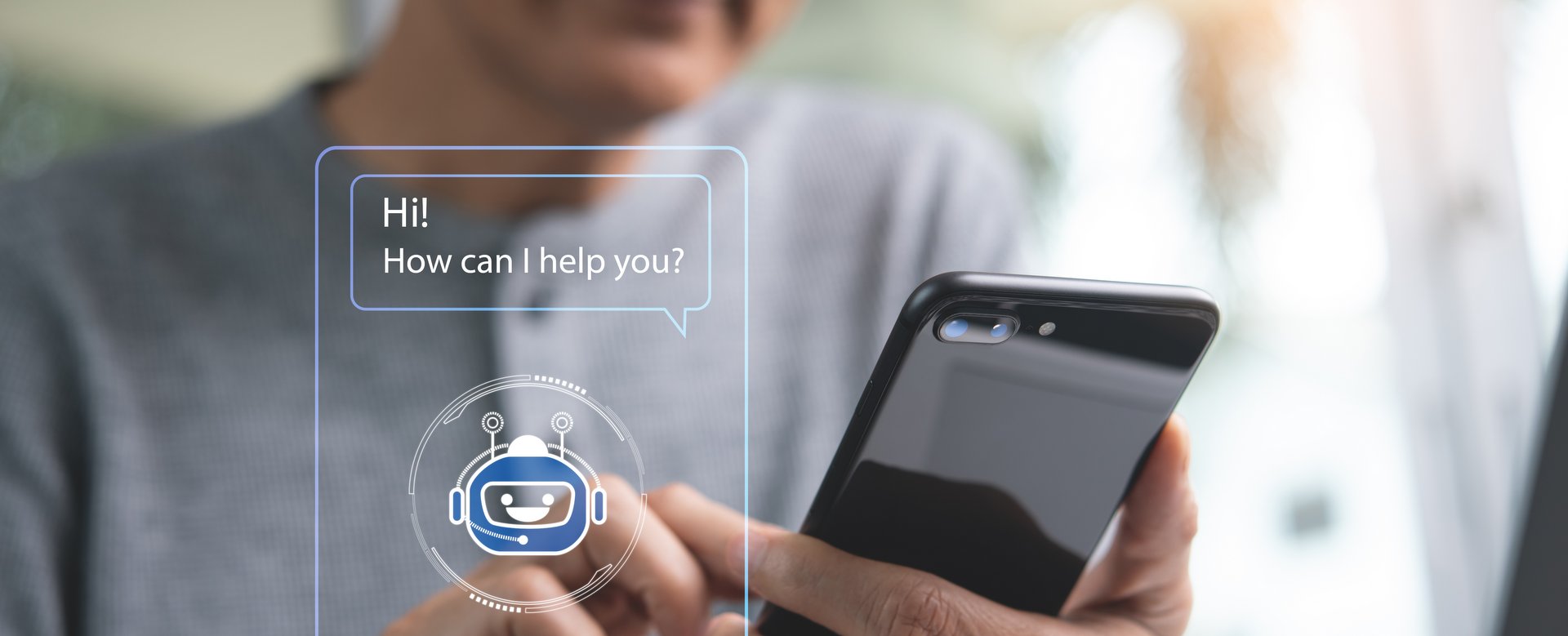
Enhancing customer experience managment by bringing together AI, Engineering, and Design
The Big Picture
Our client is one of Europe’s leading media, entertainment, and communications business, serving a multi-million customer base and providing a wide range of products across television, broadband, and mobile telephony. As the entertainment industry moves towards a more customer-centric and value-driven approach, enhancing customer experience is becoming one of the significant differentiators, especially with competitors regularly offering discounts to gain market share.
To address this challenge, Fractal recommended a framework driven by AI, engineering, and design, to understand the customer experience management in real-time and identify its key drivers. The solution enables upstream decisioning and designing of marketing intervention strategies, thereby proactively reducing the propensity to churn and reduce the number of cancellation calls. The solution also allows the company to avoid being pressed into rolling out expensive retention offers at the time of renewal, nurture a more value-based relationship, and drive long term value with customers.
Transformative Solution
By working closely with multiple teams within the client setup, including customer management, products, campaign management, information technology, insights & analytics, and customer service teams, Fractal developed a solution to transform the client’s analytics ecosystem and drive more proactive customer experience management decisions. The key benefits from the recommended solution include:
- Ability to define a value-based relationship and help the organization to move away from price discounts, and offer-based retention, towards a long term, continuous experience-based approach leading to better net promoter scores and higher value per customer.
- Deep understanding of drivers of customer experience to help design customer-centric strategies and proactively improve relevance and expertise across the customer lifecycle.
- An easy-to-implement relationship score metric (0-100) to provide a holistic view of customers’ entire relationship with the business – across products, interactions (online and offline), evolving customer needs, and more – so it works as a single source of truth and is consumed organization-wide.
- Built the first customer experience management solution on the Google Cloud for the organization resulting in accelerating their move from on-premise data and technology platforms. This involved transformation of tool-sets, end-user training on GCP, development of GCP compatible algorithms, building data pipeline for live data feeds to cloud, and connecting the outputs to marketing platforms for consumption.
The Change
The solution enabled ongoing identification of customers with the highest propensity to churn through a leading ‘proxy’ – customers showing signs of a degrading experience with the brand, which enabled our client to arrest the issues earlier and achieving significant savings (reduced cancellation calls, contact center costs) and reduction in value erosion due to churn. Upstream targeting of just top 2% of customers at risk week-on-week translated to:
- Call reduction: 7-10% reduction in the total annual volume of cancellation calls
- Churn reduction: 5-6% reduction in the total annual volume of churn
- Revenue-loss prevention: Arresting average drop in subscription revenue per customer/quarter, leading to $40M+ in value savings in the first year
- Cost reduction: $20M+ savings due to the reduction in cancellation calls and churn prevention activities
The Implementation
Key to success was bringing together AI, engineering, and design:
Artificial Intelligence
- Model architecture: A merged deep learning architecture was built on GPU-enabled distributed data processing cloud architecture to track and predict the customer experience 1-3 months into the future. The algorithm captured both relatively static information, such as demographics, recent product holdings, pricing, and billing., as well as dynamic data, such as the sequence of historical transactions/interactions (customer journeys), which were critical to understanding the future churn behavior.
- Adaptive learning: Based on the response to targeted treatments (captured in incremental data) as well as new trends emerging due to various business initiatives and external factors, the deep learning framework continuously auto-learned and recalibrated to provide accurate estimates of future customer experience, without any need for manual intervention.
Engineering
- Data harmonization: The engineering module performed customized data transformations on terabytes of raw data, coming from over twenty different sources, at varying levels of granularity, into a single source of truth for the entire customer base. The data captured information on customer product holdings, demographics, and inbound/outbound interactions, along with customer/client-initiated transactions at a weekly level. It generated a holistic view of every customer across multiple dimensions and formed the foundation for developing the deep learning framework.
- Acceleration: Process automation, parallelized data processing, and modeling resulted in efficiencies at scale and delivered a time reduction of 20X compared to traditional frameworks.
Design
- Building a ‘simple to measure’ metric: Given the requirement for the score to be consumed across multiple teams and functionalities within the client organization including marketing, contact center agents, technical engineers, campaign operations, customer management, etc.—it was critical for the metric to be intuitive and easy to interpret. The solution was thus designed to generate a score for every customer every week, ranging between 0-100, where lower values indicated poor experience and vice-versa.
- Building a compatible data science environment on the client’s platform (Google Cloud): GCP provided an ideal environment for optimal data processing performance in a distributed manner on the full customer base in terms of time and platform cost. Two separate multi-node clusters were set up to handle variable workloads of data harmonization/engineering and model training/scoring, respectively. The deployment of the deep learning algorithm also followed architecture native to Google Cloud, ensuring maximum efficiency and minimal deployment cost. Below is a holistic view of the GCP tools/services that were leveraged during the solution design and development:
- Simplifying consumption of the deep learning framework: Model outputs were consumed by the business stakeholders via an interactive dashboard, which was used to monitor the health of the customer base via a high-level view of the evolution of the experience scores, as well as monitoring the performance of the customer segments decision and other critical business metrics. This also served as a single source of truth across the organization.
- Modularized framework enabling scope for future enhancements on GCP: The framework is highly modularized to allow future enhancements or alternatives in terms of tools/services as and when released on GCP. For example:
- Using Cloud Dataflow for data processing/harmonization: The Cloud Dataflow provides a fast and simplified pipeline for large-scale data harmonization. It provides a distributed auto-scaling framework, leveraging serverless processing without any complex workarounds.
- Machine Learning using AI Platform: Tensorflow based distributed deep learning functionality on AI-platform enables usage of huge data for model training while Tensorboard allows real-time tracking of model performance during training.