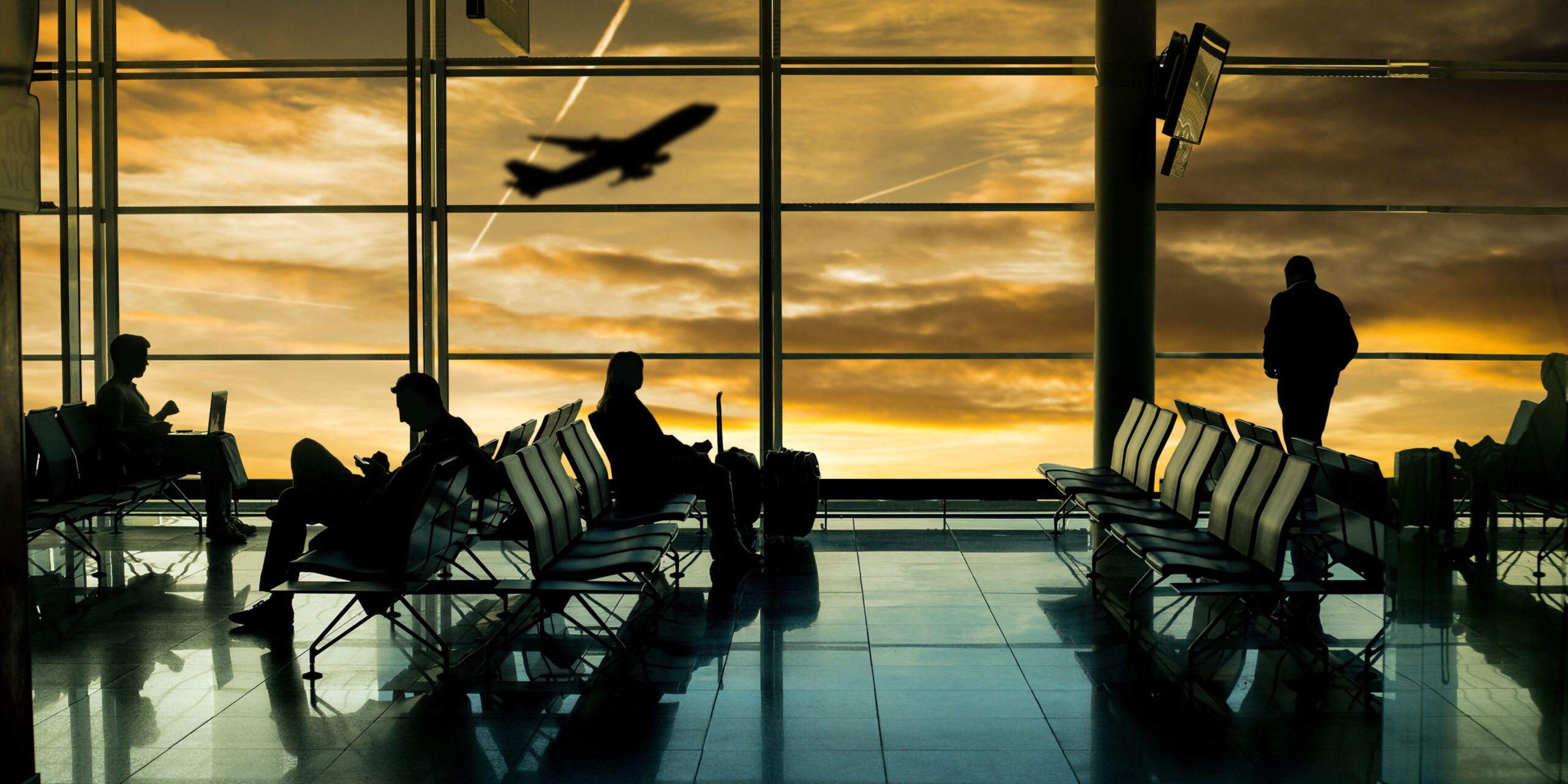
Fractal’s Solution Improved Bag Predictions for a Top U.S. Airline
Summary
Carry-on luggage is the biggest bottleneck when it comes to boarding. There is pressure on gate agents to plan the boarding process during pre-flight meetings effectively. To combat this, a major U.S. airline, known for its extensive domestic and international operating network, sought to revamp its carry-on estimation system.
Accurate carry-on estimates are crucial for airlines to manage cabin space effectively, ensuring enough overhead bin space for passengers. Additionally, knowing the number of bags helps airlines allocate appropriate boarding time and implement strategies for smoother boarding in case of higher-than-expected luggage volume.
The existing system faced challenges like data limitations, inaccurate predictions, deployment complexities, and low user adoption by gate agents. This hindered the airline’s ability to manage cabin space and boarding times efficiently.
To address these issues, a solution was developed that integrated various data sources and built advanced prediction models that delivered real-time information to gate agents. This involved focusing on feature engineering and modeling experiments to improve accuracy and address under-prediction issues. Additionally, the solution was deployed on AWS and included a user-friendly application displaying a range of bag estimates instead of single-point predictions. This comprehensive approach significantly improved the model’s predictive power, empowering the airline for smoother boarding and better passenger experiences.
Challenges
The customer had a rudimentary model to estimate the number of carry-on bags using historical flight data, future flight schedules, active bookings, passenger details, and checked baggage data. This solution was deployed on a non-rigid architecture that used batch processing and did not incorporate real-time data or feedback. This created several challenges that impacted its ability to efficiently manage cabin space and boarding time.
These challenges included:
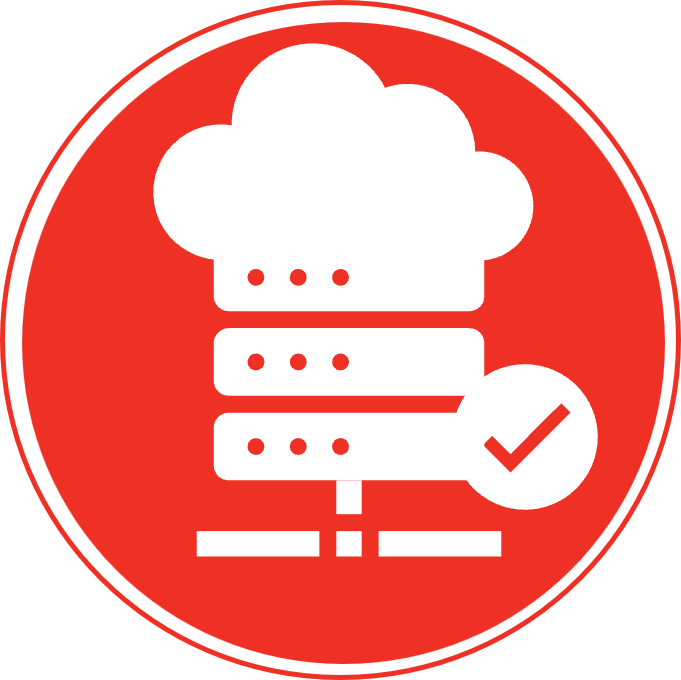
Data availability
There were issues with the availability of real-time data, such as the real-time check-in count and the monitoring of available cabin space.
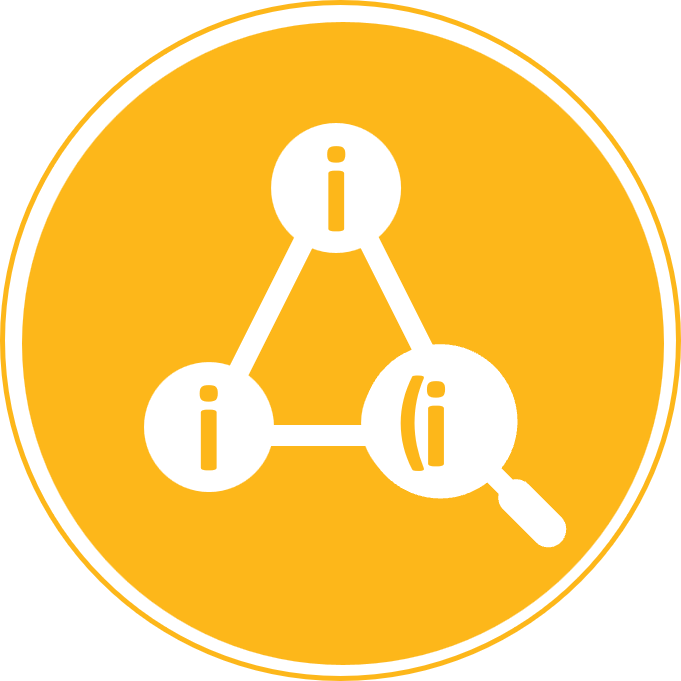
Prediction errors
The predictions made by the client’s model were not accurate, leading to dissatisfaction among the gate agents.
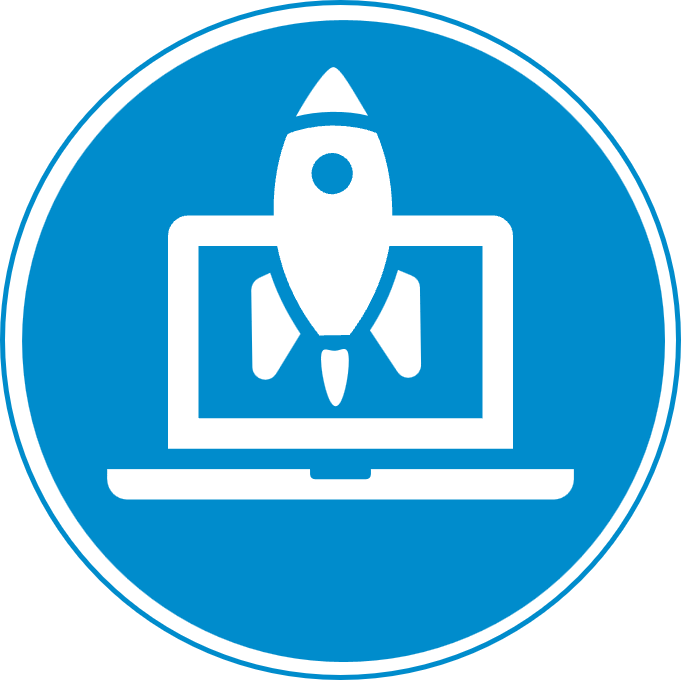
Model deployment
The client’s model encountered challenges in fine-tuning and updating due to its non-rigid architecture and the absence of real-time data.
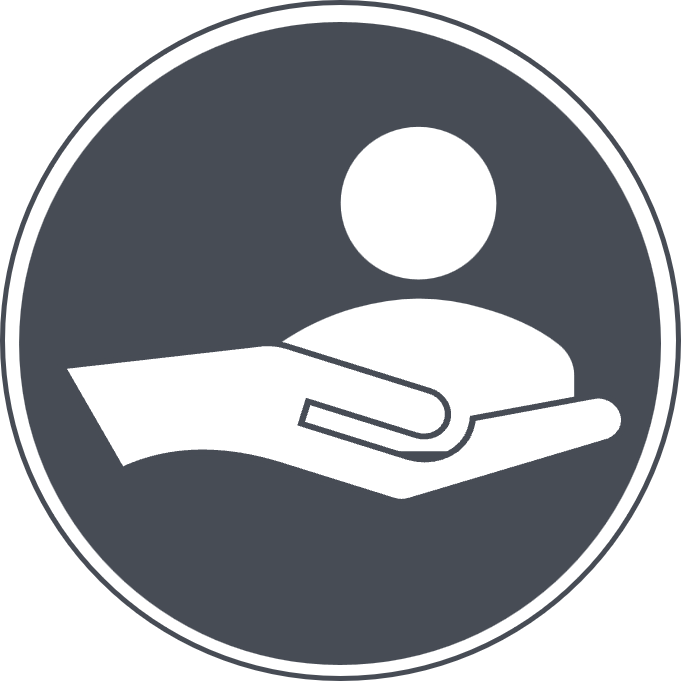
User adoption
The agents were reluctant to implement the client’s model because the client model lacked clarity and precision in its predictions.
Solution
Fractal used a systematic approach that integrated different information sources, built predictive models using CatBoost Regression, and brought real-time information to the gate agents. Fractal also developed feature engineering, feature selection, and modeling experiments to improve the accuracy of the predictions and reduce the under-prediction issue.
Fractal deployed the solution on Amazon Web Services (AWS) and created a front-end application that displayed a range of gate bag estimates instead of point predictions. The solution helped identify flights with a higher likelihood of delay and triggered proactive handling of gate bags. The also solution improved the predictive power of the model, enhanced user trust and adoption, and reduced the flight delays caused by gate bag handling.
Results
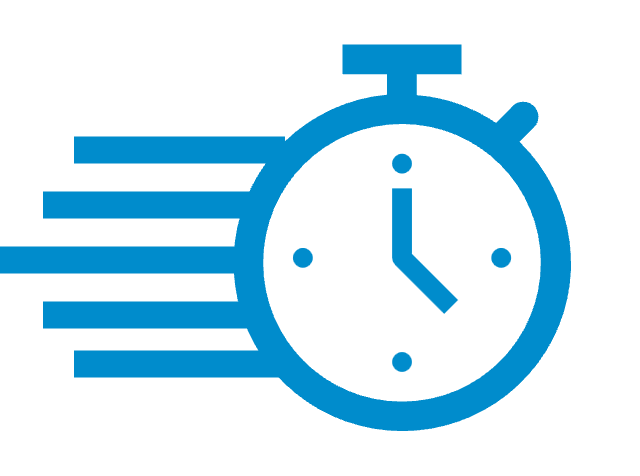
Immediate Benefits (Post-Implementation)
- Improved Predictive Power: Our solution enhanced the existing machine learning model, accurately forecasting gate-checked bags.
- Reduced Flight Delays: Gate agents’ increased adoption of our tool significantly minimized overall flight delays.
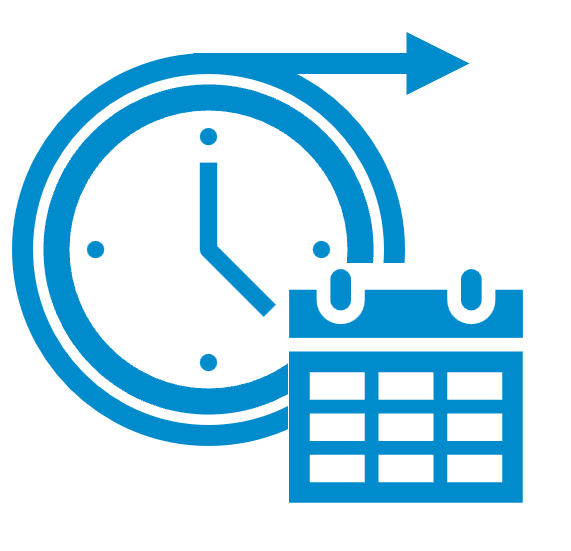
Long-Term Benefits
- User Adoption: End users embrace the robust model, benefiting from near real-time gate bag predictions.
- Operational Efficiency: The constant feedback loop ensures optimal baggage handling and enhances passenger experience.
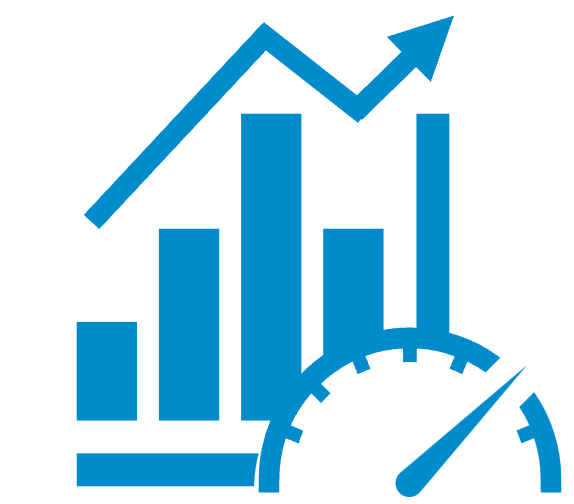
Key Performance Indicators (KPIs)
- Model Accuracy: Achieved an impressive 90% accuracy in recommendations.
- User Adoption: End users and gate bag agents actively utilize the solution.