From Inspection to Automation: The Role of Machine Vision in Modern Manufacturing
Authors
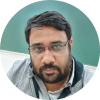
Prosenjit Banerjee
Principal Data Scientist | Machine Vision and Conversational AI
Machine vision is revolutionizing the way companies manufacture. It transforms supply chain and logistic systems into smart cyber-physical systems that can extract vital information remotely through images and videos.
Until recently, the major applications of machine vision in manufacturing were on condition or process monitoring systems. In the early days, the motive was to reduce dependency on
Exceptional progress in vision feature engineering coupled with steep rise in deep learning and camera sensor technology, have opened a plethora of opportunities across the manufacturing and supply chain, which earlier had existed only in theory.
human operators by installing a camera strategically in a manufacturing unit to capture continuous events or sequences as images/videos. These unstructured data were processed with state-of-the-art machine vision algorithms to infer the criticality of events in real time and augment knowledge to an operator in his decision-making process. This led to the development of automated optical inspection (AOI) systems which could detect defects of manufactured units in a production line non-intrusively, removing the faulty units to land in delivery. The introduction of ethernet server-based systems enabled vision sensors to stream / store, process, and infer from large volumes of data. It was now possible to interconnect multiple vision systems and make quicker inferences, enabling faster decision-making. Suddenly, the production lines became faster, with informed or scheduled downtimes and significantly fewer manufacturing errors. Simultaneous advancements in camera /vision sensor technology and machine vision algorithms scaled the adoption of vision sensors towards other related events in manufacturing like situational awareness in factory floors, regularise human intervention in critical or hazardous areas, maintaining safety standards in production like adherence to helmets, gloves, PPE kits, detect an early outbreak of fire, prevent accidents and better asset management.
The growth and adoption of cloud technology and IoT fostered investment by the manufacturing industry toward digital platforms. With the history of production knowledge and decision-making, replicating or repurposing manufacturing systems became the need of the hour. The concept of digital twins was introduced as an analytical environment for closed-loop decision-making for any known entity in a value chain, thereby connecting decisions from the strategic to the operational level. Machine vision has supported the realization of digital twins, from creating virtual environments for production to the virtual commissioning of machines.
Machine vision generates compelling business value among early adopters. However, as Gartner states, the highest-value machine vision solutions are hard to adopt, replicate and scale. McKinsey pegs the number at 72%, where organizations have tried to adopt yet met with less success. How do we then harness the power of machine vision applications? How do we architect adaptable and scalable machine vision solutions?
Machine vision has always delivered significant value within uniform parameters or working conditions. The difficulty in scaling machine vision solutions in some sectors may be attributed to the lack/absence of standardization in their processes. This may require additional investments where legacy systems may have to be upgraded to meet required standards. Other roadblocks towards machine vision adoption are witnessed in data privacy, where data about critical processes were absent in model building.
Machine vision enhancing manufacturing efficiency
Machine-vision-powered manufacturing and supply chain solutions have been driving improved production efficiency across different industrial sectors. In recent years, machine vision and cloud computing have enabled the usage of Augmented Reality/Virtual Reality.
Virtual commissioning of machines through simulation assists in rigorous testing of production parameters, without actual usage of machines. This has resulted in reducing the cost of procuring expensive machines and cutting down costs by huge margins.
Production line quality check
Replicating production process
Bulk manufacturing, customization & packaging
Integration with robotics
Addressing the top 3 challenges
Manufacturers still have apprehensions when it comes to adopting machine vision solutions. However, consistent advancement in this domain has been addressing their concerns.
Halting production
Challenge: To ensure seamless transformation of production lines, where defects or concerns are addressed without halting production or impacting any loss in product volumes.
Solution: State-of-the-art depth cameras enable quick scanning of machine parts, reverse engineering, editing changes, printing fresh designs, and putting processes back to production in almost no time. This ‘non-intrusiveness’ of machine vision technology enables its adoption into existing processes without halting or causing significant changes in the production line.
Scaling production
Challenge: There is an urgency to replicate or scale up successful machine vision solutions. However, the transition may not be smooth as requirements and models may vary.
Lack of data
Challenge: Lots of data flows into manufacturing units but may not be retained or labeled. Where success highly depends on the quality of data available, the absence of labeled data or no data is a major hiccup while delivering solutions to the manufacturing or supply chain industry.
Solution: Machine learning, a part of machine vision, now develops models that work on less data footprint. Where thousands of images were earlier required to train models, now with semi-supervised learning methods, models can be fine-tuned with very less or partially labeled data to generate effective solutions.
Fractal initiatives in different sectors
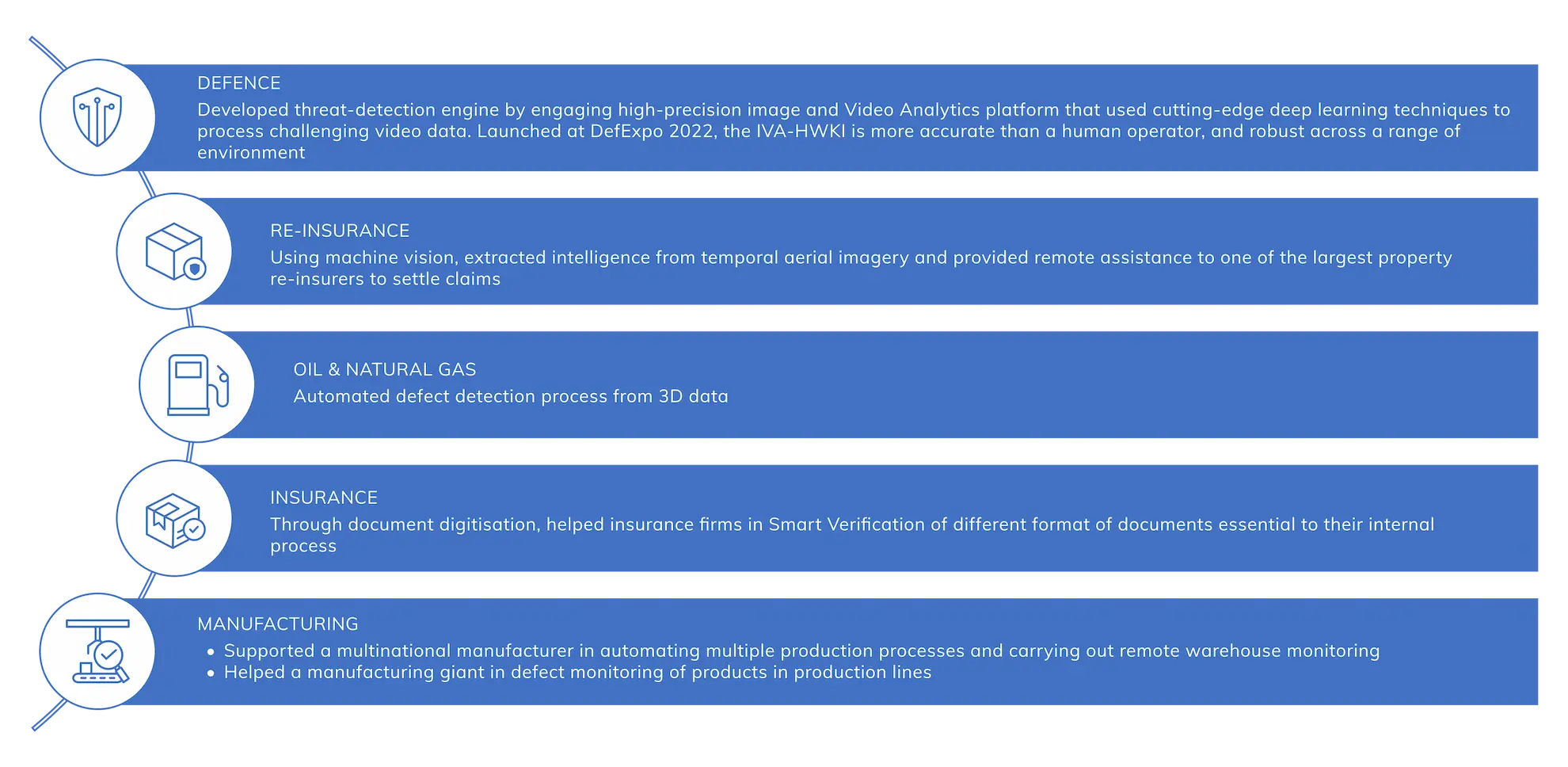
How Fractal tackles data privacy issues
Data privacy is a big concern. The fact that the data can be reused and models repurposed is a cause of constant worry among stakeholders.
Fractal has a dedicated machine vision team focusing on efficient solution deployment. In most cases, the hardware rests with third parties. But once the data flows in, the team builds models with limited annotated data to provide state-of-art solutions.
A large part of the solution is then deployed on the client’s device, followed by regular updation of the model. More and more of the solutions are being moved to the Edge to strengthen privacy further. With this technology, data can be processed closer to where it is generated. Organizations prefer solutions where a major part of data resides on their devices. Fractal has been working in federated learning for some time now, and our solutions always have an aspect of privacy preservation factored in.
Fractal Edge
Fractal has a team of self-driven and enthusiastic professionals from premium Indian institutes. While freshers bring in their enthusiasm, experienced professionals bring along their domain knowledge. The machine vision team has been built over the years. Today they carry the capability of in-depth research, putting the research into production, and delivering effective solutions.
Way forward
Intensifying research, advanced machine learning engineering, and progress in deep learning are fuelling the growth of machine vision. Technology is still expensive, but personalized and customized solutions can soon be a norm, enabling smaller manufacturers to afford them. The future will see manufacturers accelerate digital transformation through machine vision solutions and achieve new accuracy, efficiency, and quality levels.
- Share this post