Helping enterprises solve data-related problems with Generative AI
Authors
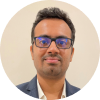
Milind Jadhav
Principal Data Scientist, AIML – ADM
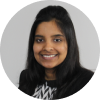
Harshitha Parsi
Senior Data Scientist, AIML – ADM
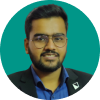
Varun Bhargava
Data Scientist, AIML – ADM
Principal Data Scientist Milind Jadhav, Senior Data Scientist Harshitha Parsi, and Data Scientist Varun Bhargava break down GANs, their emerging applications, and Fractal’s industry-leading developments.
Advances in Generative Adversarial Networks (GANs) — and the synthetic data they create — are fast-tracking intelligent, data-driven decisions that drive businesses forward.
Data drives decision-making in business processes, services, and products that move the global economy forward. However, despite the volume of real-world data available, at the enterprise level, the datasets may need to be more extensive or more openly available for use to derive relevant insights.
The business case for synthetic data
Enterprises need augmented data to solve analytical use cases where real data is insufficient or unavailable. Existing data is copied or sampled until the data set is large enough to produce results. This method creates oversights that can lead to inaccuracy and data compliance issues. Synthetic data addresses many of these problems.
Accuracy
Instead of just adding repeated records to a data set, using synthetic data to augment the scarce real data leads to more accuracy in replicating the underlying joint data distributions. This is because synthetic data creates fake/synthetic entities or customers based on the behavior and parameters of real-world data, leading to the generation of more representative data overall.
Data privacy
Many countries have introduced data protection and privacy legislation. While this does not necessarily prevent a business from using customer data, there are tight controls to ensure it is used only for the reason it was collected. Synthetic data negates this issue: it imitates the properties of the real personally identifiable information (PII) data to ensure accurate analytics, but as the data is not linked to a real-world entity, it can effortlessly be created, shared, and disposed of without compromising data subjects or contravening privacy laws. This makes access to relevant data faster and easier.
Cost
The acquisition, processing, and analysis of external real-world data are expensive for most enterprises. Once a generative model is in place, the cost of generating new data drops, making it more affordable.
Development cycle
Prototypes and innovative ideas require rigorous testing to ensure market relevance. Synthetic data enables the opportunity to test and demo products in various iterations, shorten the development cycle and increase market speed and product adoption/success rates.
GANs: From basic to advanced
Generative Adversarial Networks (GANs) are a machine learning framework designed and introduced in 2014. GANs consist of two neural networks, a generator, and a discriminator, which compete against each other in a zero-sum game. The end goal is to train the generator to produce data so accurately that the discriminator can no longer identify it as fake. Once sufficiently trained, the generator can synthesize accurate data at scale.
Initial developments in GANs focused on generating images, but the past few years have seen substantial literature around their ability to rate tabular enterprise data. This has opened the door to diverse applications across many industries.
Fractal: Taking GANs one step further
Working with enterprise data across multiple domains such as consumer banking, retail, insurance, and telecom, Fractal has a rich experience in customer-centric data. Since most data enterprises collect is tabular, we realized that the need to generate synthetic tabular data outweighs other unstructured data types like images or audio.
When we started our research, the idea was to address the cost, privacy, and scarcity challenges organizations face with tabular data.
We divided our approach into four phases and completed the first two. In the first phase, we created a synthetic data generator utility that generates a single data set of static features with no temporal element. This includes anything that does not change over time, such as demographics, gender, or occupation. The output is a synthetic version of static data inputted.
Of course, not all data is static, but it can also include time-series features. So, the second phase included creating synthetic data from real data features such as daily/ weekly/ monthly number of transactions on a credit card or activity on a website. The temporal element we built in the utility can now simultaneously account for both static customer data and variable monthly data.
Our Experimental Results
Synthesized data must accurately represent actual data to produce relevant insights. We discovered that real data sample sizes as small as 10,000 samples are enough to train our architecture to generate synthetic data sets of up to a million unique, accurate records.
Currently, there is no universally accepted single metric to measure accuracy, so we have created a custom metric based on Mutual information data matrices calculated on real and synthetic data. We followed it up with MAE calculation on these matrices to arrive at a single 0 to 1 score, closer to 0, indicating synthetic data is remarkably similar to real data. Along with this custom metric, we also use qualitative metrics such as PCA plots, TSE plots, correlations, and distribution comparisons to see univariate distributions of features in the real data and check that the counterparts in the synthetic data were similar. In both phase 1 and phase 2 iterations, our model outperformed traditional GANs.
Data set: Churn data set- bank attrition data with information about the customer demographics and transaction amounts at a customer level.
Samples: 10,000 samples
Features: MAE values for 20 features across various experiments show improvement in performance from left to right:
Experiment
VGAN
WGAN
CTGAN
Fractal data generator
MAE
0.38
0.36
0.2
0.1
Experiment
MAE
VGAN
0.38
WGAN
0.36
CTGAN
0.2
Fractal data generator
0.1
Univariate comparison plots:
Fast and sophisticated
At their core, GANs are a marriage of two neural networks, making them twice as difficult to train as single neural networks. Once training begins, the correct combinations of hyperparameters are essential to producing high-quality, accurate outputs at the end of the process. Hyperparameters like the number of layers, number of neurons in each layer, learning rate, optimizer, and architectural decisions of using the right GAN variant are critical to tune to the specific input real data provided.
As GANs are still in their infancy, we discovered that many data science users might need more technical knowledge of deep-learning algorithms to generate high-quality data. To address this, we consolidated our extensive research on which GAN works best for specific data sets into a utility that guides users. Acting as a “plug-and-play” code accelerator, it is designed to automatically select and use the parameters that will produce the best results based on the original input data. Using these recommended parameters generated synthetic data incredibly quickly and with good similarity to real input data.
For highly technical users who want complete control of the GAN setup, the Fractal utility provides maximum flexibility to adjust every individual parameter of both the generator and the discriminator. The ability of the Fractal model to cater to users across a wide range of technical skill sets makes it one of the most sophisticated GANs capabilities currently available.
Future applications of GANs
We are entering phase 3 of our development, exploring methods of generating multiple data sets in one go without compromising the inter-relationships between them. Our developments have significantly advanced Fractal’s capability in the Generative AI space. We are optimistic that our existing and prospective clients will benefit tremendously from this offering and further solidify Fractal’s position as a leader in AI research and solutions development in the wider analytics industry.