How knowledge graphs are the answer to better decisions for your business
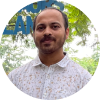
Sanjeev Kumar
Senior Data Scientist
AI @scale, Machine vision & conversational AI
Knowledge graphs are increasingly powering AI applications today. Yet, for scalable implementations and solving enterprise data integration challenges, leaders must take an agile approach to knowledge graph development. According to Gartner , by 2024, enterprises using knowledge graphs and semantic approaches will have 75% less AI technical debt than those that don’t.
Knowledge graphs can solve multiple data integration challenges that are still barriers to AI adoption related to data complexity, quality, and accessibility. With knowledge graphs, there is a fluid data environment using uniform identifiers, flexible schemas, and triples instead of tables.
However, there are varied perspectives and interpretations of what a knowledge graph constitutes. Data management focuses on the creation, use, and representation of metadata. Knowledge engineers see knowledge graphs as a concept of domain understanding, and business users look towards the hidden insights that can be surfaced by utilizing specific links and data relationships.
Let’s find out how knowledge graphs are the answer to better decisions for your business.
Using knowledge graphs
A knowledge graph is a semantic network of three components – nodes, edges, and labels. Nodes are represented by anything – people, places, objects. The nodes are connected by edges which signify a relationship between the nodes. We assign a label to the edge which defines the meaning of relationships. These labels are part of the ontology that drives a knowledge graph’s schema.
Businesses use knowledge graphs to link and integrate data (most of which exist in silos) and form multiple interconnections. The data from various sources -complex or simple, structured or unstructured – get organized.
Knowledge graphs give businesses a bird’s eye-view of the entire data, allowing them to capture interesting insights and establish relationships between entities involved, which would have otherwise been difficult to envision before.
Taking advantage of the potential collective intelligence, knowledge graphs are being used by:
- Pharma & Life Sciences: For drug discovery
- Financial Services: To detect frauds and better investment decisions
- Manufacturing & Electronics: To ward off risks and improve investment analytics
- Manufacturing: To optimize production lines and supply chains
- Search & Chatbots: For recommendations and question answering
How Fractal integrates knowledge graphs for better business decisions
While Fractal has used knowledge graphs to solve client use cases in the past, it has also supported enterprises with its knowledge graph initiatives.
The organization employs knowledge graphs built by assimilating knowledge from multiple heterogeneous sources and condensing it into a knowledge graph. Fractal helped the organization build the infrastructure for data engineering, which hands over the transformed data to NLP engines for extracting the triples (building blocks of a knowledge graph).
Fig 1: Fractal knowledge graph initiative for life sciences company
How Fractal is ahead of the curve in client servicing through knowledge graph solutions
- Cost-effective fraud detection for a top British Commercial insurance firm
We detected and identified multiple frauds involving solicitors, doctors, repairers, and claimants, who were then handed over to Special Investigative Unit. This led to 40% savings on SIU’s efforts to manually go through every claim.
- Detection of tax evasion and non-compliance for an Indian Government entity
Fractal identified potential cases of tax evasion and non-compliance for an Indian Government entity. The approach was to identify entities with cash deposits, not in line with their income using pattern matching.
With our solution, we identified incremental cash information for non-PAN accounts using fuzzy matching. We also recognized Shell companies, Benami properties, cash splits, and other such patterns using link analysis and pattern mining. This helped to establish high-risk fund flow channels in a large, connected set of accounts.
- A leading pharmaceutical company in the US
Based on the members’ profiles, past purchases, and conditions, we identified pharmaceutical items for upsell.
- Customer acquisition, expansion, and segmentation in a major UK-based CPG enterprise
Fractal built a Consumer 360 knowledge graph related to company purchases. This helped the organization find loyal customers across different brands and regions and enabled lead generation and customer segmentation.
How to build knowledge graphs
Structured data in a business has a relational database and follows an enforced schema, while unstructured data has no fixed schema. Both the data are combined to extract entities and form relationships between them. These entities and their relationships conform to ontologies that define the schema of knowledge graphs.
Ensuring data consistency and data model understanding, ontologies serve as the basis for instances of knowledge graphs. Knowledge graph development calls for a considerable investment of time and money.
Steps to build knowledge graph
Challenges faced
‘With data growth, knowledge graphs need regular updating to cover incoming data. The algorithms used to create data may be less than 100% accurate. Knowledge graphs developed out of such data may have inaccuracies that get carried forward. Over time, cumulative inaccuracies can lead to significant errors.
There is also the challenge of disambiguation, where the exact words or phrases carry different meanings, e.g., turkey can be a bird or a country. Hence, disambiguation of such data becomes necessary. Moreover, data can come in different languages, apart from being different in structure. Hence, it is crucial to translate it into one common language before further processing.
Knowledge graphs can easily capture a small amount of data. But as data scales up, they may need help to expand. Hence deploying knowledge graphs at scale becomes essential.
Charting the way ahead
Integrating data across the enterprise and supporting complex decisions, knowledge graphs will drive the next wave of technological advancement. And organizations eyeing scalable implementations to solve enterprise data challenges will adopt an agile approach toward knowledge graphs. Having said that, one must have a strong business case to create them, as knowledge graphs involve considerable time, expertise, and money.
While large organizations with excessive data can build their graphs, data-driven medium and small enterprises can opt for open-source knowledge graphs and customize them based on their use case. This gives every organization the wherewithal to develop knowledge graphs that will prove valuable in their business decision-making.