Production Yield Optimization
Autonomous AI
Reduce waste and optimize output with deep reinforcement learning-trained AI agents
What is PYO
Production Yield Optimization (PYO) is a proven Autonomous AI solution that enables manufacturers to reduce waste and optimize manufacturing output with AI agents.
Most production control systems are built for static environments. The AI agents act as intelligent process controllers that dynamically fine-tune existing control system parameters. The agents enable those control systems to adapt to the changing manufacturing environment.
Fractal designs the AI agents using manufacturers’ subject matter experts through the Machine Teaching process. The agents are trained using deep reinforcement learning techniques and custom-built AI simulator trained on real-life process data.
The PYO solution combines accelerators, best practices, and custom data science engagements to build, train, and deploy effective AI agents.
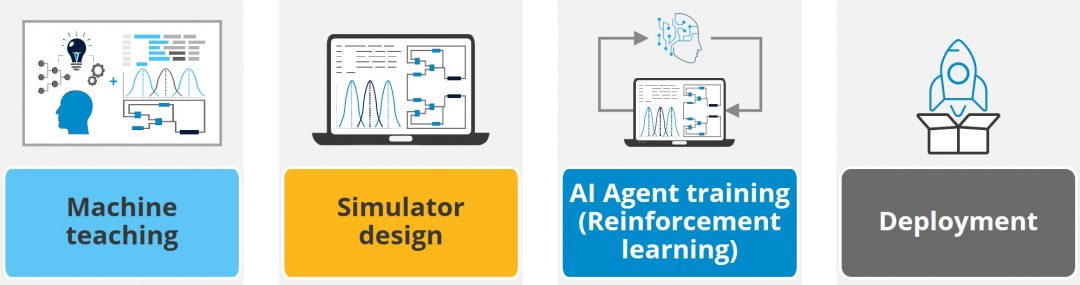
Why should you consider PYO
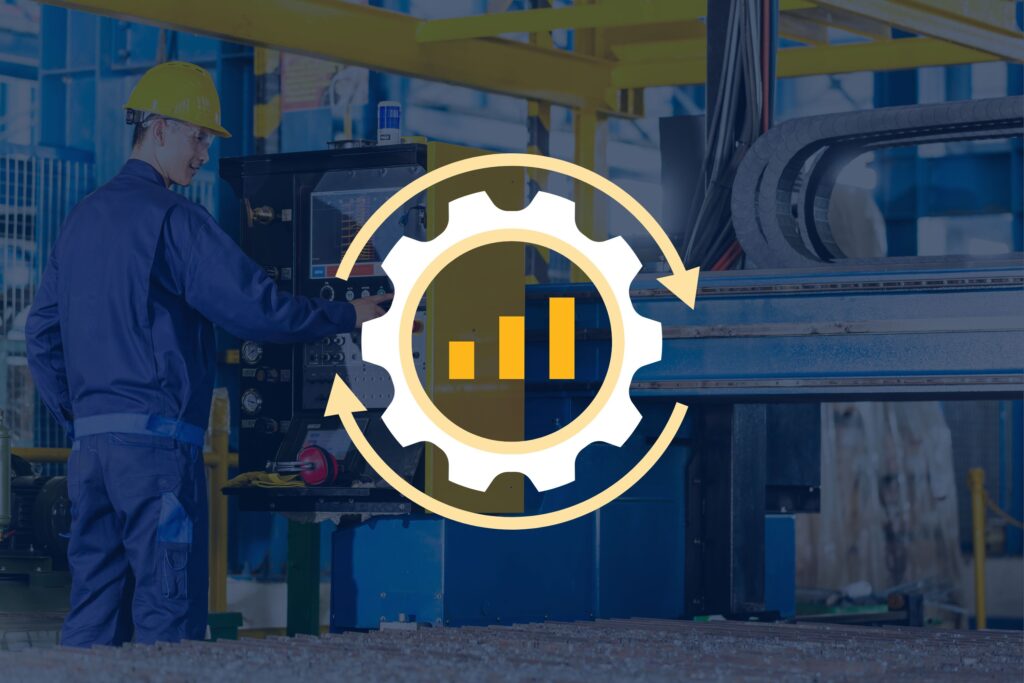
Optimize production with AI
Optimizing production to maximize yield or reduce waste and out-of-specification output is very hard to automatize. Successful optimization often relies on senior line operators and supervisors who possess an intimate knowledge of the line’s equipment and behavior. Those subject matter experts (SMEs) are a rare commodity.
With PYO, this expertise can be transferred to autonomous AI agents to help optimize production for complex, dynamic, and changing environments. Those agents will be able to tackle not only manufacturing-line level challenges such as process inputs, equipment behavior variability, or competing optimization goals but also human ones such as operator overload or talent shortage.
PYO AI agents will be trained by leveraging SME expertise and time-tested heuristics to effectively optimize production.
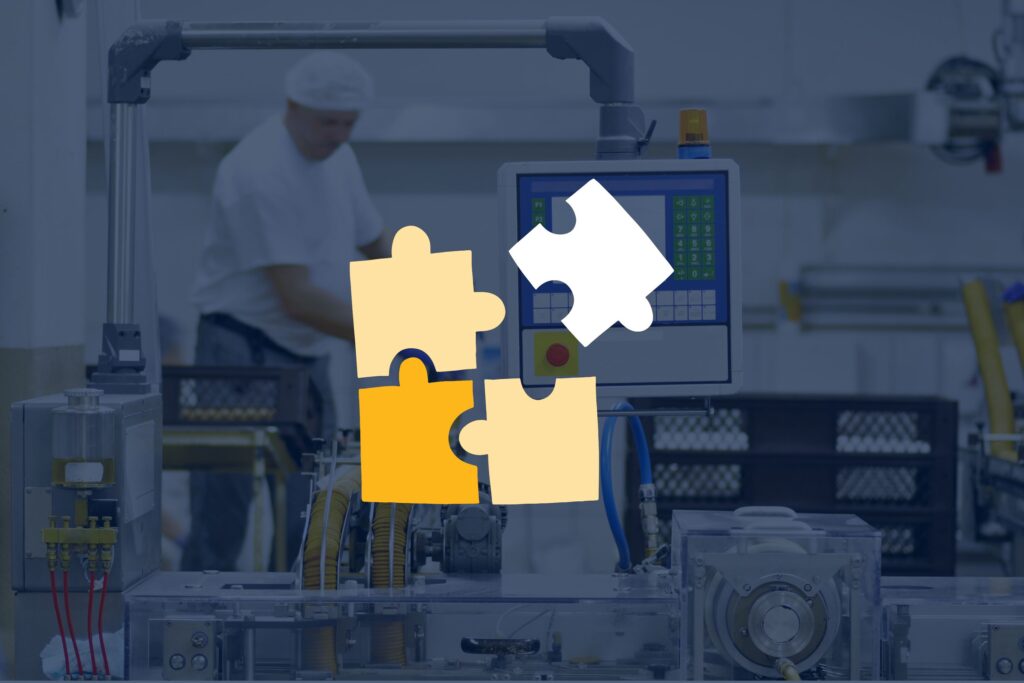
Real-world AI solution
PYO leverages deep reinforcement learning (DRL) training technology and realistic process simulations to bring the power of AI without the need for the pre-existing labeled datasets that are necessary for more traditional supervised-learning AI training.
The solution builds on the Azure Machine Learning proven end-to-end data and AI platform. It leverages industry standards reinforcement learning techniques and libraries to translate SME expertise into so-called reward functions to let the AI agent self-train with the help of the simulation. This expertise transfer is commonly referred to as “Machine Teaching.”
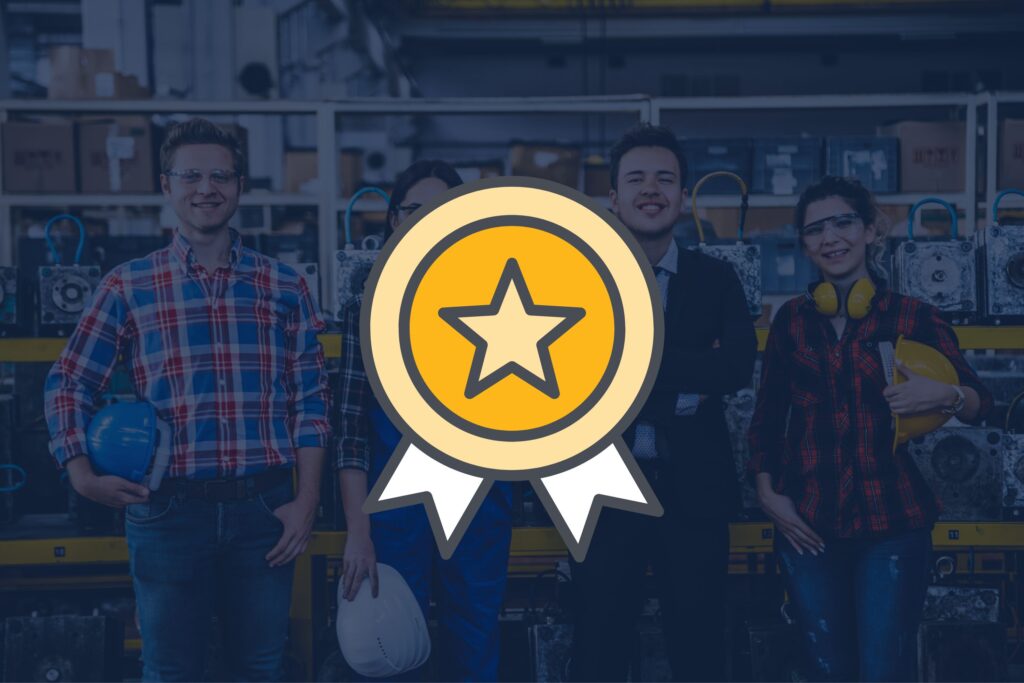
Fractal end-to-end expertise
Designing, building the required data platform and simulation, training the appropriate AI agent, testing this agent offline, and finally deploying it into live production requires a comprehensive set of skills.
Fractal brings an end-to-end DRL experience, accelerators, and best practices to help the manufacturer customize PYO to its unique needs. This is one of the key reasons why global manufacturers like PepsiCo have trusted Fractal for their Cheetos manufacturing optimization process.
Technical description
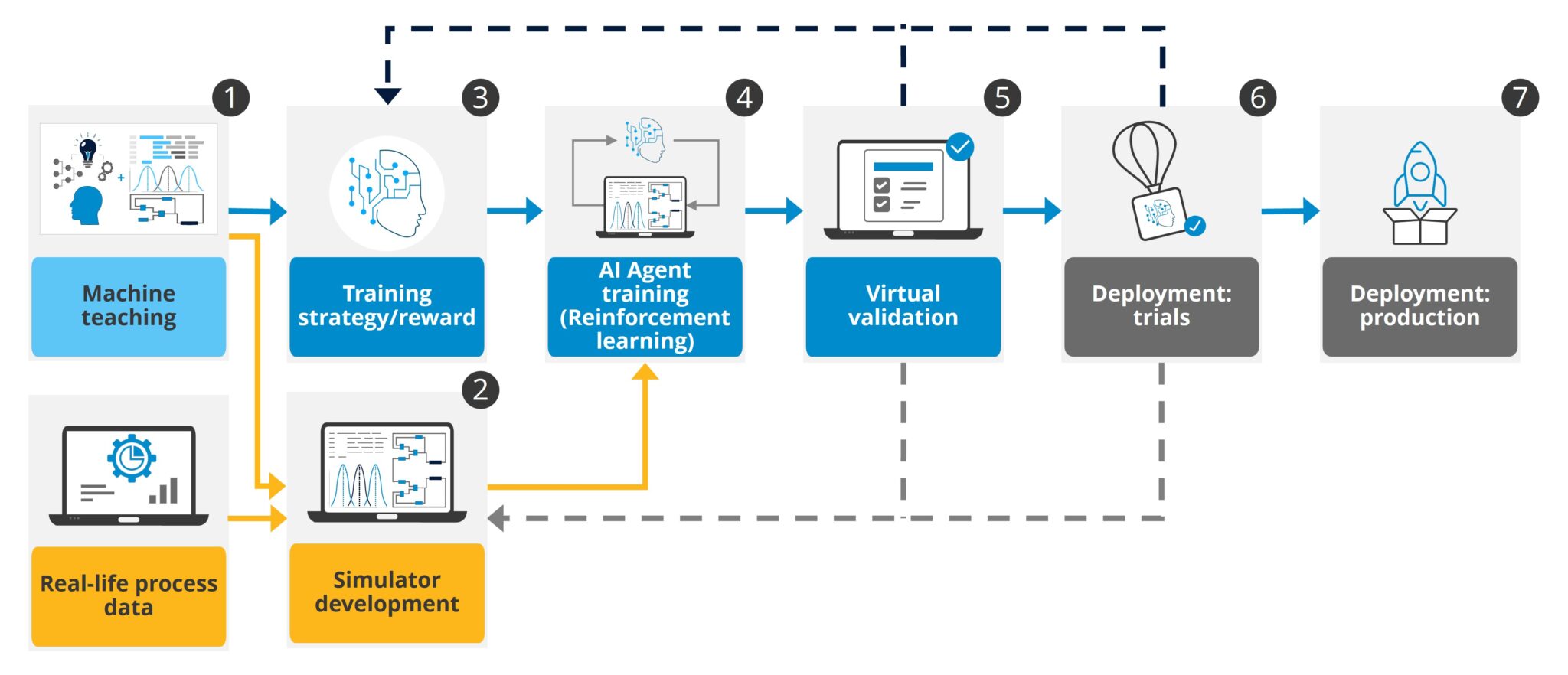
The PYO AI agent design, training, and deployment is a multi-step and iterative process.
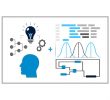
Step 1
During the Machine Teaching process, the manufacturing SMEs (operators, process engineers, product specialists, etc.) will articulate their expertise and the production optimization heuristics developed but not necessarily documented throughout the year.
They will help select the
appropriate process training
data that will be used to train the AI simulator.
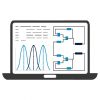
Step 2
Using real-life process data, the PYO team will develop an AI simulation closely behaving like the live process.
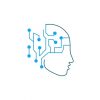
Step 3
Using the Machine Learning step insights, the team defines the reinforcement learning reward function.
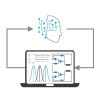
Step 4
The AI agent is then trained using the simulation.
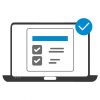
Step 5
Once trained, the AI agent
behavior is tested using the simulation.
Depending on those results, the process may continue to trial deployment or may require going back to the simulation design (2) and training strategy (3) steps.
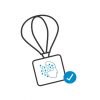
Step 6
If the virtual validation is passed, the agent can then be tested in real life under close supervision.
One option can be to let an operator decide whether an agent’s decision should be pushed to the control system layer or not versus letting the agent directly
modify parameters.
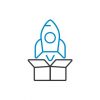
Step 7
The final step consists of deploying the agent in production either as an operator assistant, leaving the operator in charge of executing the proposed change, or as a “meta-controller” directly controlling the process controllers (e.g., PLCs) parameters as needed.
How does Deep Reinforcement Learning train an Autonomous AI agent?
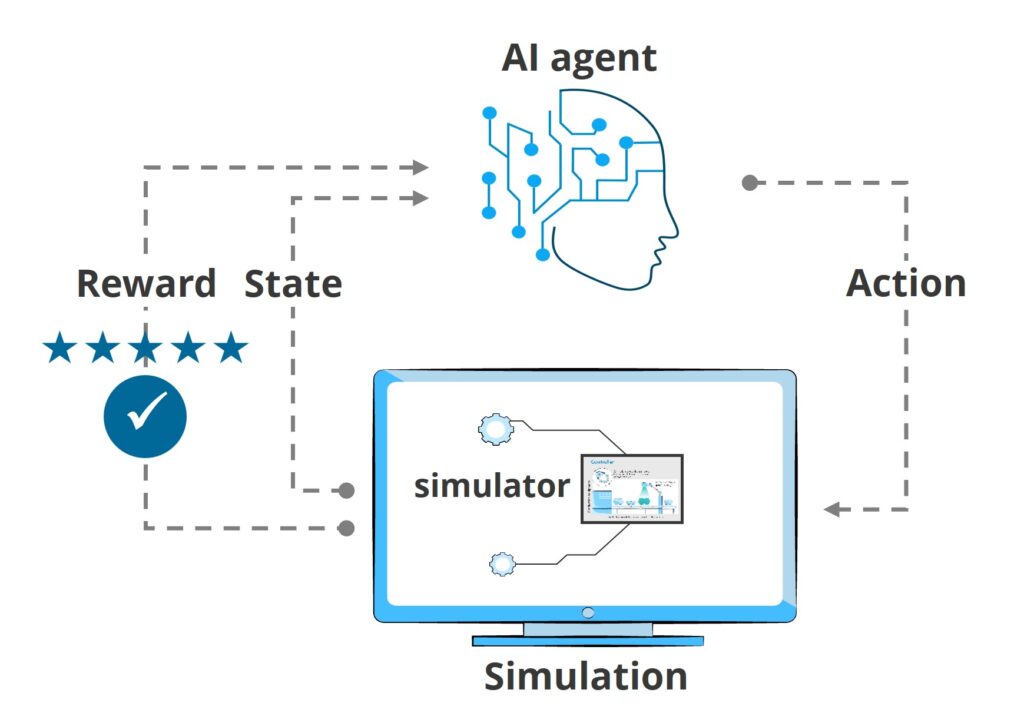
Deep Reinforcement Learning (DRL) uses the concept of a “reward function” to provide continuous feedback to the agent.
The agent sends control signals to the simulation. The reward function will measure the difference between the expected simulation state versus its actual state and will modify the agent’s deep neural network weights accordingly.
Depending on the system controlled, this training loop will run between hundreds of thousands to millions of times.
PYO Autonomous AI in action at PepsiCo’s Cheetos plant
It’s the perfect combination of human and machines!
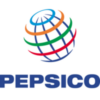
Denise Lefebvre
SVP, PepsiCo Global Foods, R&D
Note: Neal Analytics, a Fractal company that helped develop the solution for PepsiCo as described in this video, and its PYO Autonomous AI experts have been integrated into the parent company, Fractal, since early 2023.