Generative AI (GenAI) has reshaped the data analysis landscape, empowering analysts with diverse technical backgrounds to conduct data processing tasks efficiently. Beyond its influence on data analysis, GenAI plays a pivotal role in data engineering by automating boilerplate code generation and facilitating seamless data movements from source to destination. Moreover, it excels in crafting patterns for data processing, enabling engineers to direct their attention toward the intricate aspects of their work, such as coding complex transformation logic. The synergy of AI-generated patterns and custom code in this hybrid approach marks a significant leap forward in automation within the field.
Use cases in data engineering
Bridging the gap between automated data analysis and broader data engineering applications is exemplified in a case study with one of Fractal’s clients.
GenAI was integrated across the data lifecycle of a client, significantly enhancing efficiency. It facilitated table creation, data movement, and test case generation, significantly reducing time and effort. This integration automated repetitive tasks, accelerating data availability for analysts. GenAI’s ability to interpret prompts allowed analysts to handle complex data analysis tasks efficiently, boosting productivity across the board.
This functionality shows significant potential in complex sectors like finance: it streamlines tasks by automating the creation of functional data — which is crucial for testing modifications in data pipelines. For example, rigorous regression testing is essential when financial data pipelines undergo minor changes. GenAI expedites this by generating the required test data, bypassing the labor-intensive process of manual testing and interaction with sensitive production data. This efficiency speeds up the implementation of changes and enhances data security through features like data masking, ensuring that sensitive information remains protected during transfers. The result is a more agile, reliable, and efficient data engineering process.
This shift towards automating and enhancing data engineering practices in complex sectors illustrates GenAI’s broader impact, where its integration is reshaping code generation and fundamental roles within the industry.
Automating and improving code quality with GenAI
Integrating GenAI, particularly Large Language Models (LLMs), into engineering significantly transforms code quality and data engineers’ roles. LLMs are now crucial in generating reliable and high-performance code, reducing human error, and ensuring consistency across different coding tasks. A critical new aspect of this approach is establishing clear distinctions between AI-generated and human-written code, necessitating new industry practices and “guardrails” for accountability and reliability.
Fractal’s Morpheus, a GenAI-based cloud migration and rationalization workbench, exemplifies GenAI’s potential in software engineering, significantly accelerating migration and rationalization programs. It supports various migration patterns to the cloud and features an intuitive GUI and CLI interface. Its capabilities include fast-tracking development in inventory analysis and accelerating migration across various platforms. This demonstrates its adaptability in SQL, ETL, and model migration and highlights its role in leveraging LLMs for translating code between technologies while ensuring high standards of reliability and performance.
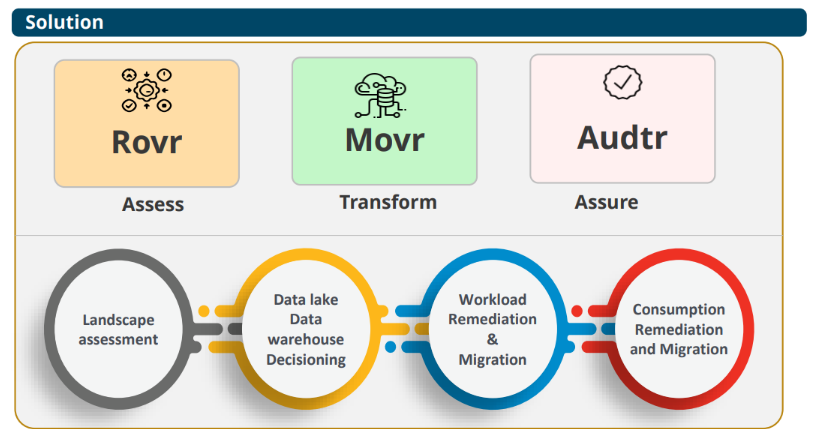
These advancements pave the way for significant efficiency improvements in test cases and data generation, overcoming traditional completeness challenges and time consumption in functional testing.
Efficiency in test case and data generation
Traditionally, achieving a complete set of test cases for functional testing was daunting, often resulting in only partial completion. This inefficiency was not just a quantitative issue but also a time-consuming one, demanding extensive domain analysis by test engineers. GenAI enables generating upwards of 90% of complete test cases, significantly reducing the time required from days to mere minutes.
GenAI’s impact extends to test data generation, a vital aspect of testing in data-sensitive fields like finance. For instance, at Fractal, LLMs have been fine-tuned to create functionally accurate test data for specific domains, such as developing models for detecting account takeover fraud. This capability of GenAI ensures the production of data that mirrors real-world scenarios without the risk of compromising sensitive information, thereby maintaining regulatory compliance and data integrity.
The use of GenAI in these processes marks a significant stride in enhancing productivity. Test engineers can shift their focus from the laborious task of generating test cases to more value-added activities like analyzing test results. In essence, GenAI doesn’t just streamline the testing process. It also elevates the quality and reliability of software development and deployment, fostering a more efficient, secure, and compliant testing environment.
GenAI’s profound impact in enhancing testing efficiency and data integrity in software engineering seamlessly transitions into a distinct impact in business intelligence and analytics.
Advancements in natural language processing and querying
The implementation of GenAI is changing how data is analyzed and interacted with. This transformation is evident in enhancing business intelligence (BI) dashboards and reports. GenAI automates complex data analyses and interprets data to provide in-depth insights into emerging trends and anomalies. This automation extends to creating and using machine learning models, enabling efficient generation of these models, or leveraging existing models from organizational repositories. This feature significantly reduces the time and resources required for model development, thereby streamlining the analytical process.
One of the most significant advancements brought by GenAI is in natural language querying. Historically, translating natural language queries into specific code patterns, such as SQL, especially for intricate queries involving multiple tables and complex data operations, was a daunting task. GenAI technologies have overcome these challenges by enabling more contextually aware and accurate prompts. Advanced pre-trained models like GPT have expanded natural language processing capabilities, allowing for robust translation of user queries into executable commands.
Fractal’s Crux Copilot embodies this revolution in BI. It offers a conversational interface that allows users to interact with and query data like natural conversation. The platform can answer complex ‘What,’ ‘Why,’ ‘Where,’ and ‘How’ questions, providing personalized insights and narrative summaries. Crux Copilot’s anomaly detection and pattern recognition capabilities enhance its utility, making it a comprehensive decision intelligence platform. Its adaptability in integrating various data sources and platforms, including AWS, GCP, and Azure, showcases its versatility and enterprise readiness.
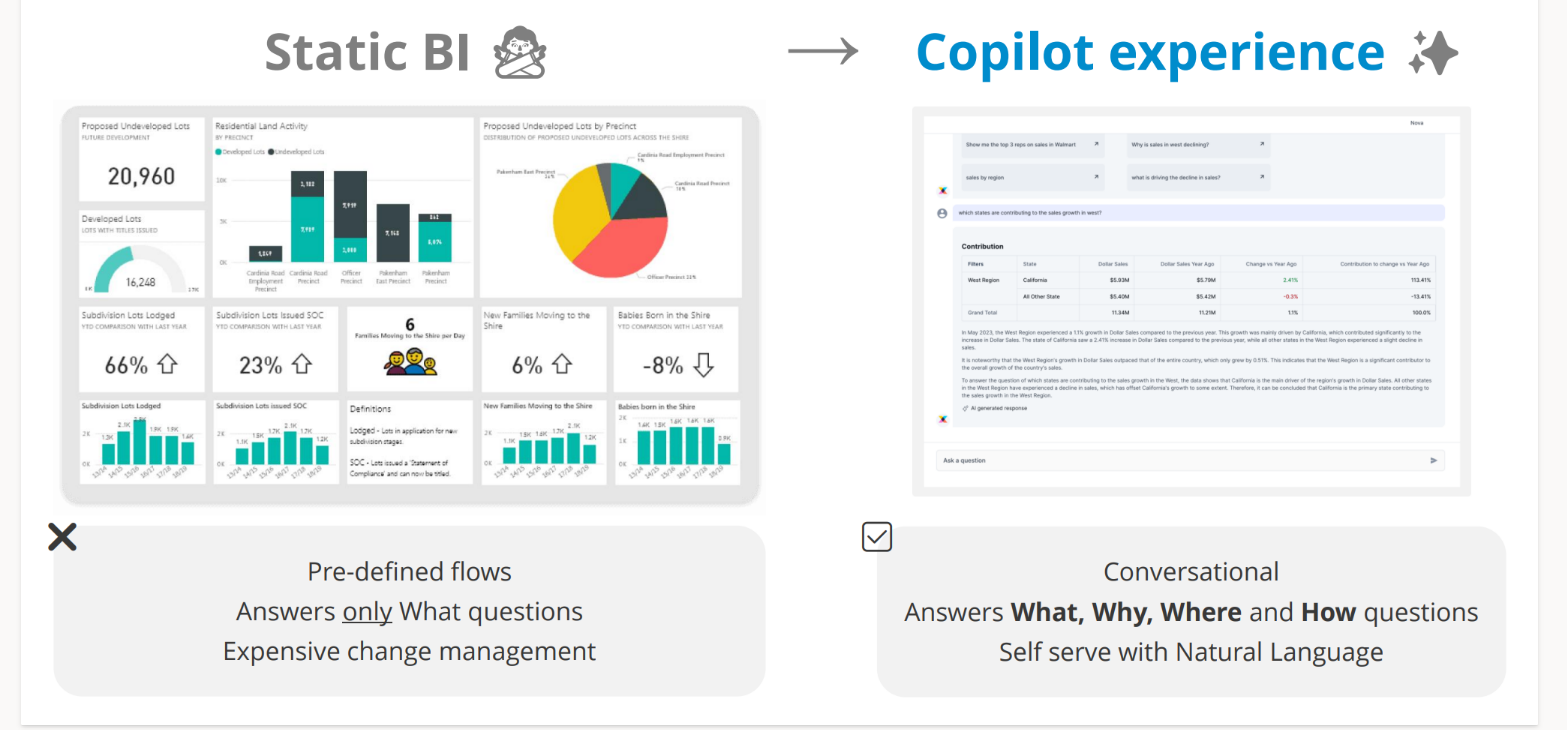
The advancements in GenAI are enhancing the functionality of BI tools and democratizing data access and analysis, making it more accessible to an extensive range of users of varying technical proficiency levels.
Future trends and potential challenges
The natural evolution in GenAI marks a significant shift towards more intelligent, responsive, and user-friendly data analysis in the business world rather than simply technological proficiency. These advancements have streamlined complex technical tasks and fostered innovation and efficiency, enabling professionals to concentrate more on addressing core business challenges. This paradigm shift allows for a more business-centric approach, where the primary goal is developing solutions that directly impact business outcomes rather than just overcoming technological obstacles. This change increasingly emphasizes the importance of innovatively solving business problems.
GenAI has advanced data engineering toward a more streamlined future. From automating complex processes and ensuring efficient data pipeline testing to enhancing security measures, it empowers data engineers with tools like Morpheus for efficient cloud migration, embodying a shift in roles and responsibilities within the industry. This shift to a more intelligent and responsive approach in BI and analytics, coupled with democratizing data access, underscores GenAI’s transformative impact on business problem-solving and technological innovation.