Upcoming industry trends in AI
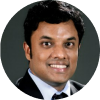
Suraj Amonkar
Client Partner
AI@Scale, Machine Vision and Conv. AI
Artificial intelligence (AI) has come a long way in the past two decades. However, it wasn’t until Google’s Attention Mechanisms paper was published in 2014 that the wider community began to realize its true potential. Since then, we’ve seen the rise of large language models, and the latest iteration — GPT-4 — is a significant inflection point in AI’s journey.
Despite the latest developments, however, there are still several challenges facing AI and its relationship with humans. Addressing these complex issues is likely slower and more difficult than advancing the technology itself. But with the global impact, AI promises to deliver; it’s becoming essential to tackle these challenges with the same vigor we’ve shown thus far in evolving AI.
The simple/complex dichotomy
The evolution of AI seems to be going in the opposite direction of human evolution. Animals prioritize survival, vision, and speech over more complex tasks, yet AI has already tackled some of the more complex problems and is now moving towards mastering natural language processing (NLP) which, in many regards, is less complex. While some primitive capabilities that animals possess may be harder to achieve, we are still progressing toward generalizability, which is the holy grail of AI.
With the advent of deep learning, we’ve built more generalized models capable of object detection, sentiment analysis, and topic identification. This generalizability is the breakthrough researchers and developers have been striving for, but there is still a long way to go, as AI can still hallucinate and get simple things wrong (e.g., tests on GPT-3 have proven it responds with both correct and incorrect answers).
Current AI needs to be fine-tuned to ensure that the solutions to simple tasks aren’t overwhelmingly complicated. Although the complexity of AI’s world model makes this challenging, with continued effort and advancement, we are slowly inching toward true general AI, with more distinct human-like qualities and abilities.
Not quite human… Yet.
One of the biggest questions asked regarding where AI is headed is whether its complexity will eventually rival that of the human brain. Our ability to create mind maps to link seemingly unconnected ideas is an intriguing concept that begs the question: can machines develop similar capabilities?
Although natural language processing and computer vision technologies have advanced significantly, they still lack a fundamental understanding of the interrelatedness of ideas. This is especially evident in scientific knowledge — machines currently can’t understand complex concepts such as gravity and space-time. While some research has explored embedding knowledge graphs into models, the absence of a comprehensive understanding of concepts and their relationships is a significant challenge AI faces. In the immediate and medium-term future, human intervention will still be required to ensure that AI produces accurate and appropriate results.
The question we should be asking in the longer term is not whether AI will replace humans but how much human intervention will be required for a specific task. As Fractal CEO, Srikanth Velamakanni, puts it: “rather than AI replacing humans, we might see humans using AI replace humans not using AI.” Currently, that seems to be where things are headed, which leaves two distinct challenges:
The bias, ethics, and responsible AI dilemma
There are fundamental issues with AI that still need to be resolved, such as biases in the models themselves. To make these issues even more complicated, there are differences in societal biases worldwide. The most extreme forms of bias, such as violence and discrimination, are already being addressed, but usually only after the model has been built, which is not an ideal situation. As a global community, we should be striving to improve AI by incorporating ethical considerations into the AI development process itself.
It’s challenging to determine how much bias should be removed from a model, and what kind of bias should be addressed. Should a model be completely unbiased, or should it reflect the biases present in society? These are complex questions with no simple answers.
At Fractal, we prioritize responsible and unbiased development. We have established a specialized AI ethics team composed of data scientists, data engineers, lawyers, and designers to ensure ethical considerations are considered when building our models. We have already started incorporating privacy-preserving features into our solutions — especially in the field of machine vision.
Imminent disruptions
The field of AI is moving quickly, and we are likely to see many process innovations and fundamental breakthroughs in the near future. Life sciences, healthcare, and insurance are particularly exciting areas for these kinds of developments right now. Developers are exploring the possibility of using GPT-like models, such as Bio GPT, designed to break down specialist silos and create a “brain” that knows most medical information. Although this is a huge undertaking, there is a lot of potential for a solution like this in rural healthcare, where access to doctors is limited.
Video analysis is also seeing some interesting developments. Using surveillance as the North Star of vision problems, developers are considering whether it’s possible to link and analyze footage from multiple camera feeds. So, to provide a very simplistic example, if the police need to find out who stole a blue bag while wearing a red shirt, could they search through all the footage to find the perpetrator? While it’s not immediately possible — and ethical issues around privacy still need to be addressed — breakthroughs in this sector are imminent.
To generalize or specialize — that is the question.
Another area of development is generalizable and efficient learning models. There are currently different research directions, one of which is developing small models that can perform specific tasks effectively without needing a large model that does everything. Another avenue is exploring multi-agent learning, where multiple models work together and learn from each other.
Finally, progress is also being made in multimodal language models, where multiple modes of communication are incorporated into a single generative model — for example, adding machine vision to GPT-4. The next big breakthrough is potentially multimodality in vision, which is still a work in progress. At a minimum, we are several months away from seeing it.
Fractal: Taking AI into the future
One of the key things that Fractal does is approach problem-solving in a structured way using a framework called “solving today’s problem, tomorrow’s problem, and the day after’s problem.” The first category includes tasks such as fine-tuning GPT architectures and vision models and forecasting the next best actions, as these are the immediate problems that need to be addressed to make current models work more efficiently.
One of our main areas of focus is quantum computing, which could lead to an exponential change in computation.
Fractal’s NLP and Vision team works on the next generation of AI problems, such as exploring quantum machine learning for tasks like protein folding and cognitive neuroscience. This involves understanding the brain’s behavior and decision-making and has led to forming Fractal’s cognitive neuroscience team.
Another area of research is how AI models work in low-latency, low-resource environments, the aim of which is to develop models that are small enough to work on edge devices and in ambient AI environments. Finally, the team also considers embedding AI and ethics into the model rather than treating them as external considerations.
While these fundamental problems are not application-specific, the belief is that, if we can find solutions to these challenges, we can create truly disruptive technologies.
As AI continues to develop, it’s likely to spur new industries and new ways of using technology. However, it will take time for people to learn how to use AI effectively and for the technology itself to overcome its remaining hurdles. While the jury is still out on the ultimate impact of AI, we have reached a critical inflection point, and the next few years are likely to provide some concrete answers.
- Share this post