Power enterprise decisions with AI that works
Transform your organization with AI engineered for scale, designed for impact. From optimizing global supply chains to reimagining customer engagement, our AI solutions deliver enterprise-level transformations.
Empowering enterprises to overcome critical AI barriers
Empowering enterprises to overcome critical AI barriers
AI promises transformation, but scaling remains a key challenge.
We help solve the biggest hurdles.
AI promises transformation, but scaling remains a key challenge.
We help solve the biggest hurdles.
1
Scale beyond pilots
1
Scale beyond pilots
1
Scale beyond pilots
2
Compete with digital disruptors
2
Compete with digital disruptors
2
Compete with digital disruptors
3
Deliver value for AI investments
3
Deliver value for AI investments
3
Deliver value for AI investments
The Fractal approach
Integrated core proficiency in AI, engineering, and design
Integrated core proficiency in AI, engineering, and design
Our unique strategy harnesses the full power of Enterprise AI, engineered for scale and designed for impact.
A
AI excellence for enterprise
AI-powered decisions
Automated solutions
Scalable platforms
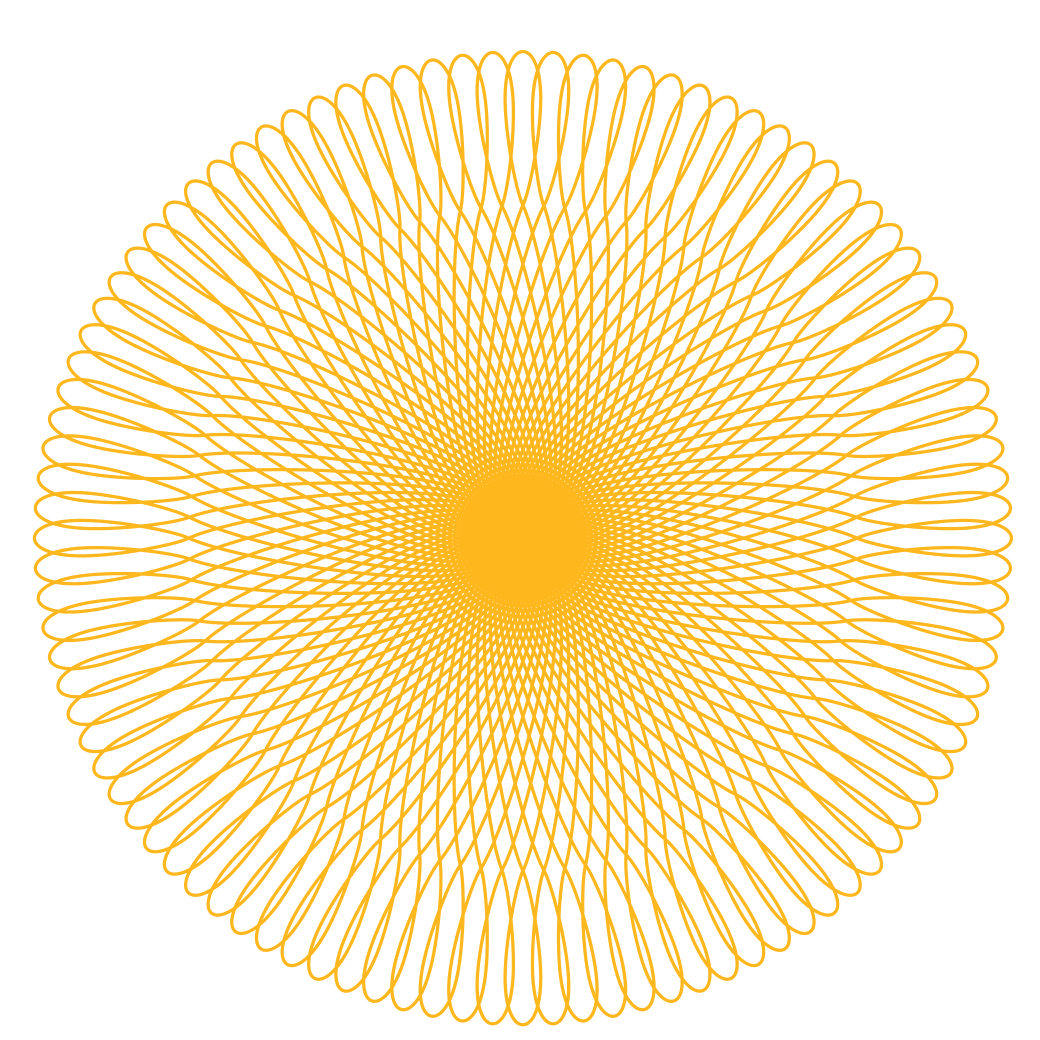
A
AI excellence for enterprise
AI-powered decisions
Automated solutions
Scalable platforms
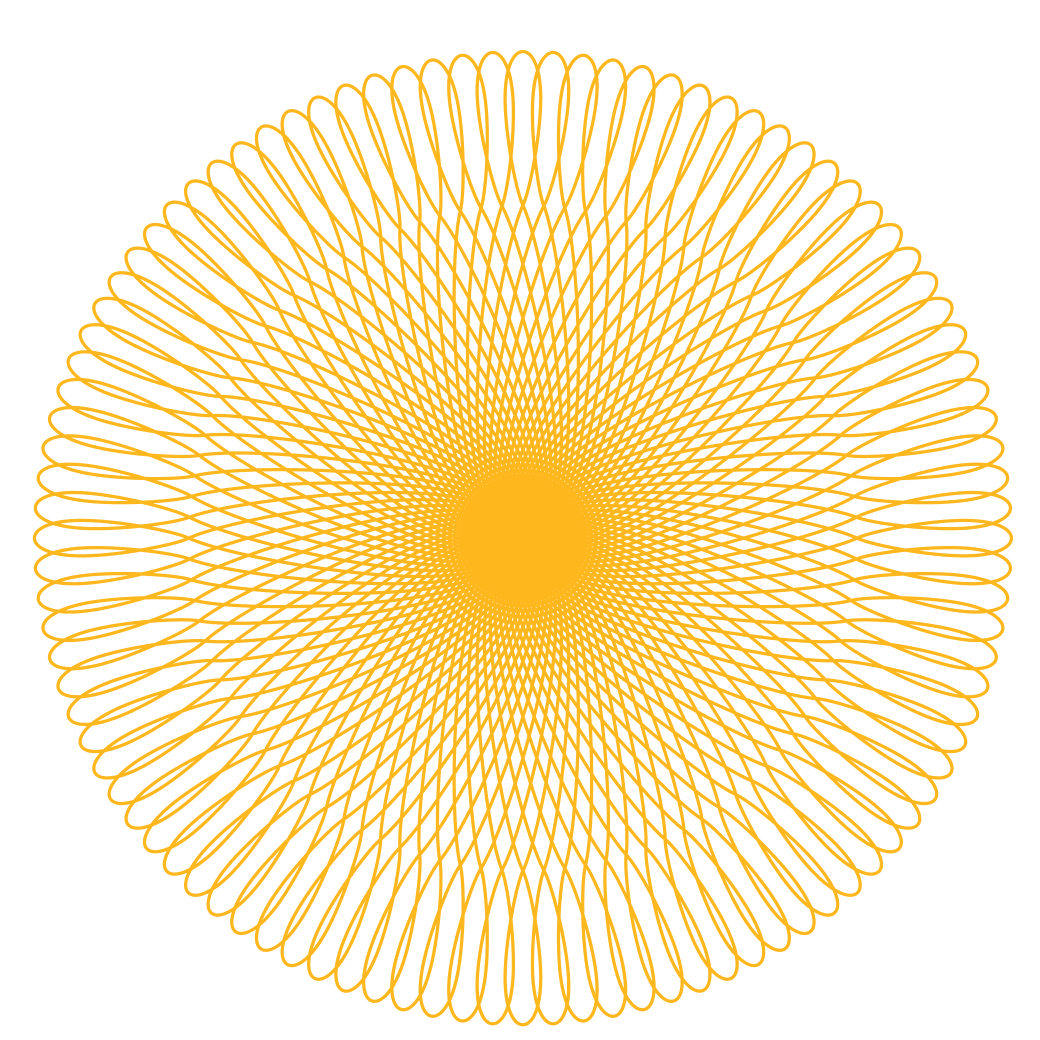
A
AI excellence for enterprise
AI-powered decisions
Automated solutions
Scalable platforms
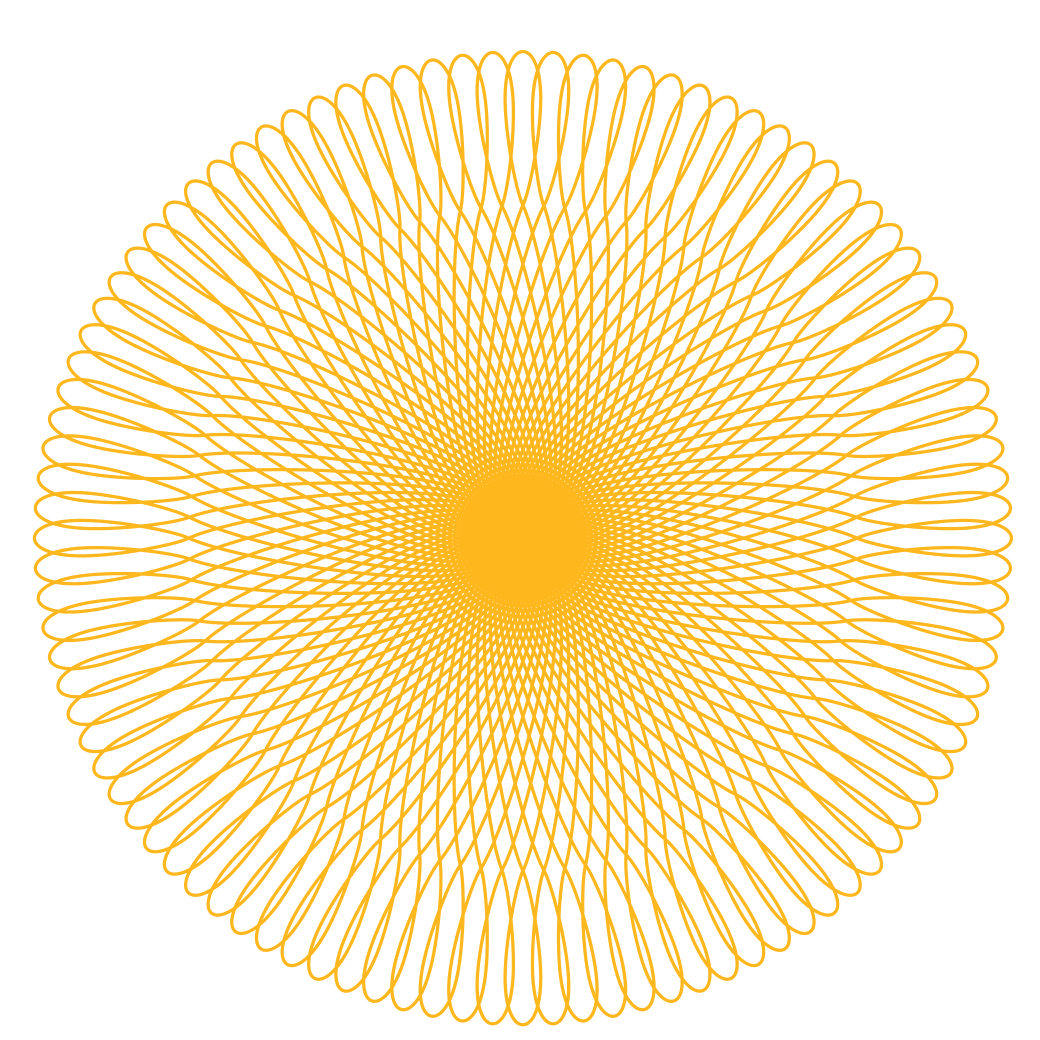
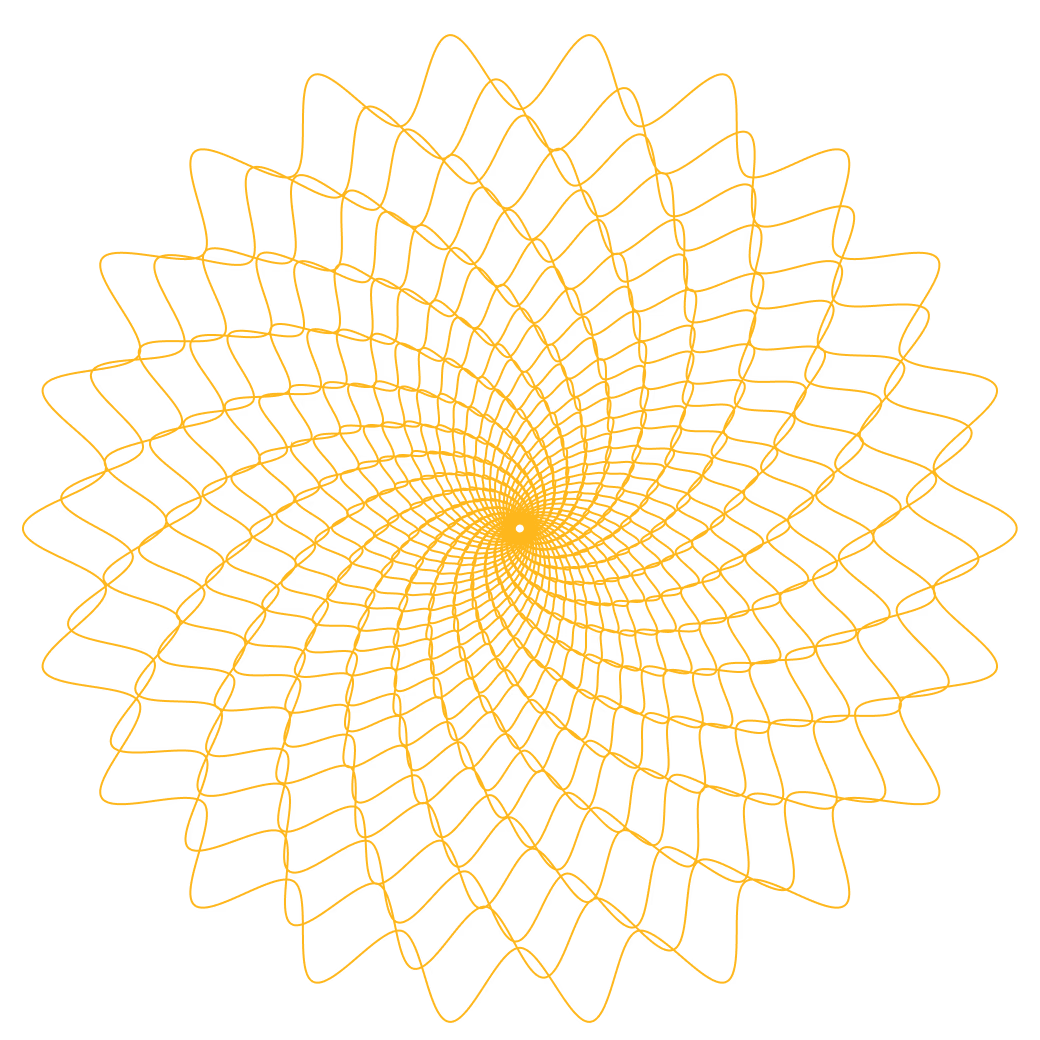
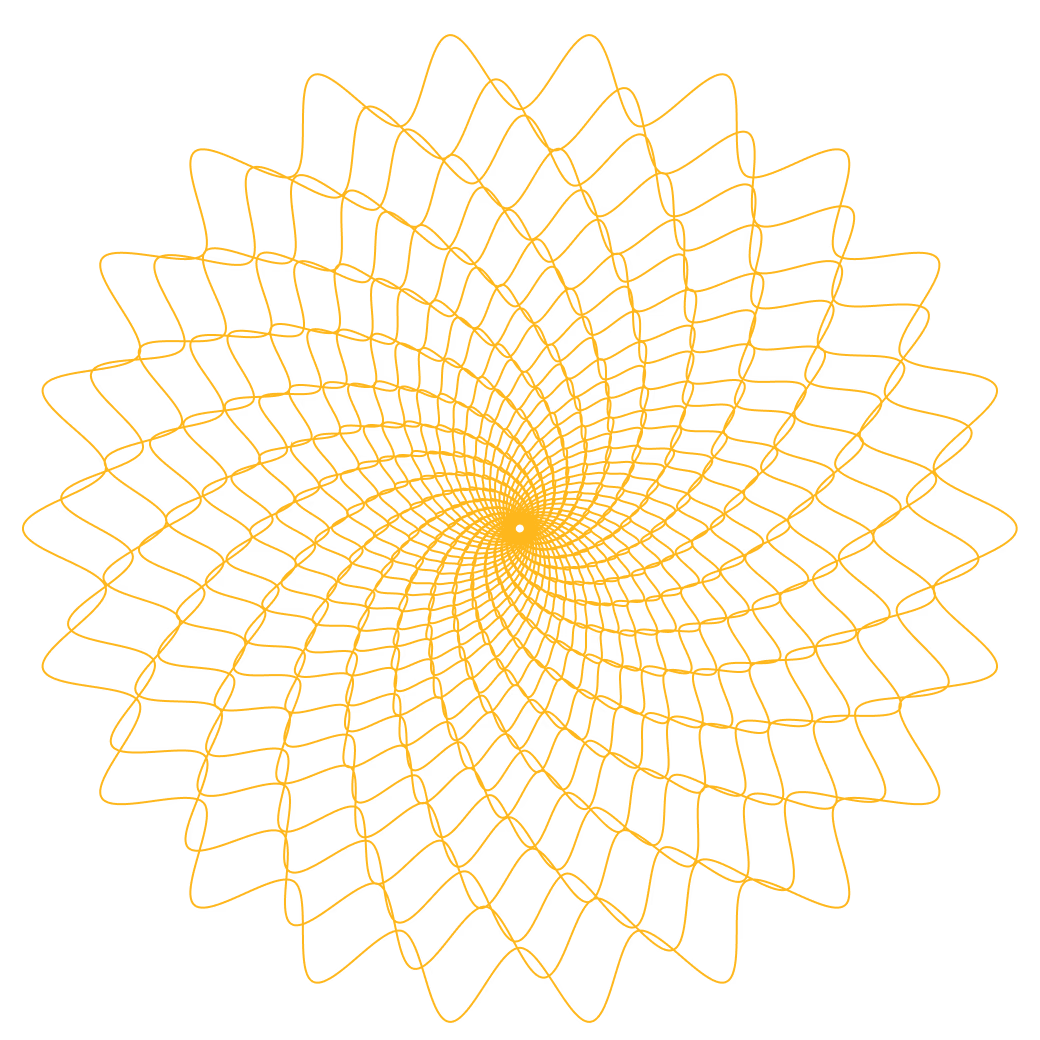
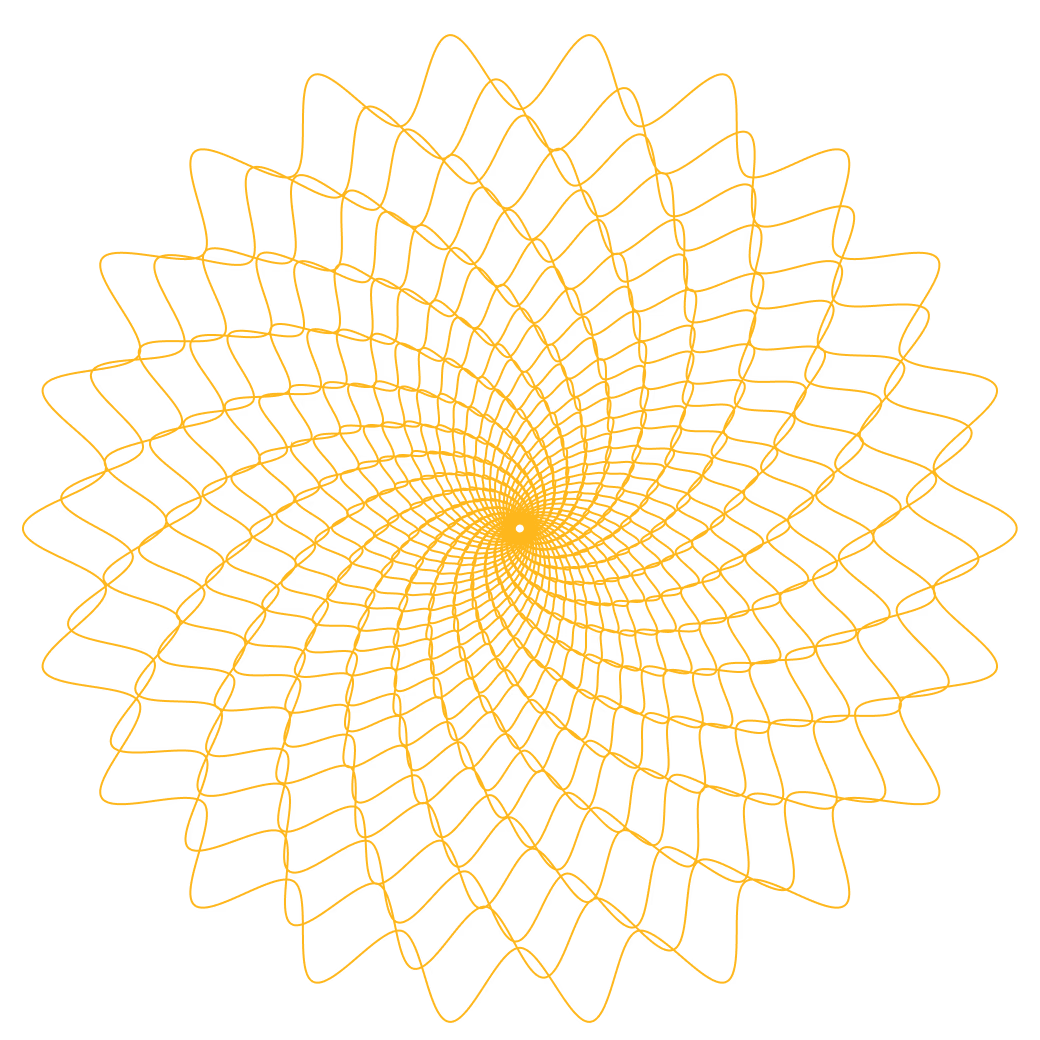



Fractal is global
Fractal is global
We are where our clients are
We are where our clients are
170+
enterprises transformed
170+
enterprises transformed
18
countries
18
countries
25
years of innovation
25
years of innovation
7%
of revenue invested in R&D
7%
of revenue invested in R&D
Industry solutions
Transforming industries with tailored AI solutions
Transforming industries with tailored AI solutions
Success stories
Building together
Great people to work with
4,600+ Fractalites embodying the best of humble, hungry, and smart

4,600+ Fractalites embodying the best of humble, hungry, and smart

A culture of innovation, empathy, and resilience

A culture of innovation, empathy, and resilience

The brightest minds dedicated to solving your enterprise’s toughest challenges

The brightest minds dedicated to solving your enterprise’s toughest challenges

A culture of connection and collaboration

Let’s transform your enterprise
Let’s transform your enterprise
Partner with Fractal to harness the full power of Enterprise AI. From strategy to implementation, we’ll help you scale AI across your organization to drive meaningful impact.
Partner with Fractal to harness the full power of Enterprise AI. From strategy to implementation, we’ll help you scale AI across your organization to drive meaningful impact.


Recognition and achievements
Select Fractal accolades

Named leader
Customer analytics service provider Q2 2025

Representative vendor
Customer analytics service provider Q1 2021

Great Place to Work
8th year running. Certifications received for India, USA, Canada, Australia, and the UK.
Recognition and achievements
Select Fractal accolades

Named leader
Customer analytics service provider Q2 2025

Representative vendor
Customer analytics service provider Q1 2021

Great Place to Work
8th year running. Certifications received for India, USA, Canada, Australia, and the UK.
Recognition and achievements
Select Fractal accolades

Named leader
Customer analytics service provider Q2 2025

Representative vendor
Customer analytics service provider Q1 2021

Great Place to Work
8th year running. Certifications received for India, USA, Canada, Australia, and the UK.
View full footer
View full footer
View full footer