At its core, Patient Analytics provides a comprehensive understanding of a patient’s treatment journey. Unlike traditional analytics, Patient Analytics delves deeper, offering insights into individual patients’ treatment journeys, from diagnosis to procedures. It does not just track sales or demographics — it provides a holistic view.
To fully grasp the context of Patient Analytics, we need to understand the three main pillars in the market. While pharma companies market to Healthcare Professionals (HCPs) as the prescribers, the ultimate consumers are the patients.
Patients | Healthcare Professionals (HCPs) | Payers |
---|---|---|
The primary consumers of the drugs | Doctors and medical professionals who prescribe the treatments | The financial entities covering the costs |
Patient Analytics uses claims data, recording patient transactions from diagnoses to treatments and prescriptions. This data provides a comprehensive overview of a patient’s medical journey, including subsequent developments like comorbidities. This constant monitoring helps healthcare professionals refine treatments and enhance patient care while also serving as a crucial tool for pharma companies to analyze the market, evaluate competition, and understand HCP prescribing trends.
Pharma’s Commercial Analytics Nucleus
Where sales data might show a doctor’s change in prescription habits, patient analytics delves into the “why” behind those decisions. For example, it might reveal adverse reactions in patients, leading to a reduced drug prescription by the doctor. This allows for a comprehensive understanding, not just of outcomes but also of the intricacies of physician behavior and each patient’s journey.
From a commercial perspective, while most data sets offer a broad overview, only Patient Analytics provides the depth needed to truly understand patient behaviors and craft personalized targeting strategies for healthcare providers. This depth ensures strategies align more closely with the unique patients that doctors see, unlocking a richer understanding of patient behavior patterns.
Tangible Benefits for the Pharma Industry
Patient Analytics has reshaped healthcare, benefiting all stakeholders, from pharmaceutical companies to the entire healthcare value chain. Take, for example, a case study we did on breast cancer diagnosis. Patterns emerged showing some patients undergoing unnecessary mastectomies.
However, Patient Analytics revealed an FDA-approved drug for early-stage breast cancer patients, which could often eliminate the need for such surgeries. By leveraging machine learning (ML), we traced pre-diagnosis patient journeys, identifying patterns like symptoms and specific tests indicating potential diagnoses. Acting on this data, we approached healthcare practitioners treating these identified patients, promoting the potential benefits of the FDA-approved drug before considering surgical options. The result was a drop in surgeries by ~30% within three months among these practitioners, resulting in fewer post-surgical complications and an improved quality of life for patients.
Beyond Just Patient Health
Patient Analytics goes beyond just enhancing patient health. For pharmaceutical companies, it provides a foundation to advocate for the inclusion of their drugs in primary insurance treatments, benefiting insurance providers, pharmaceutical industries, and patients alike. When a drug exhibits promising results, its position as a preferred choice in insurance plans can create a synergistic benefit for all stakeholders.
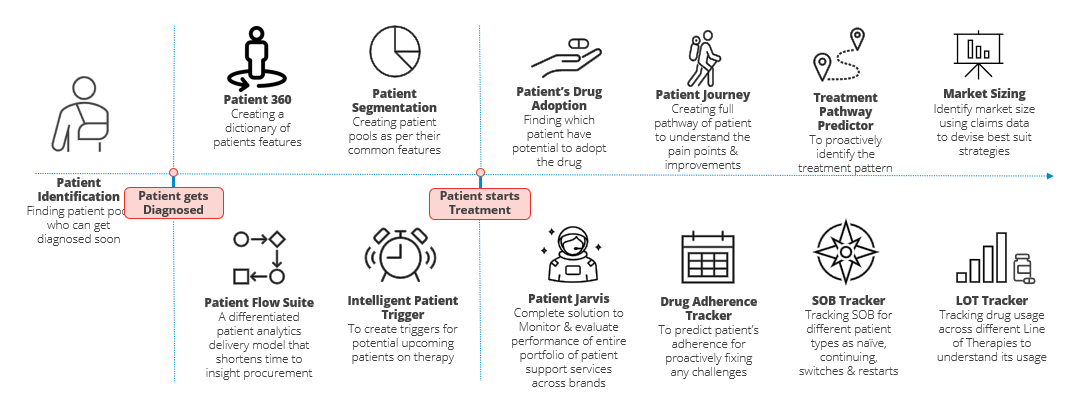
The potential of Patient Analytics is wide-reaching, as it allows for early patient identification and post-diagnosis segmentation and ensures optimal therapy delivery. We can predict treatment routes and evaluate specific market sizes by enabling intelligent responses, such as updating sales reps with critical patient data or monitoring medication adherence. Beyond mere data analysis, Patient Analytics elevates patient experiences, optimizes healthcare outcomes, and enriches the entire healthcare ecosystem, making it an irreplaceable facet of contemporary healthcare.
Challenges in Patient Analytics
Although Patient Analytics has undeniably brought revolutionary changes to the healthcare and pharma industries, it comes with its challenges, like any analytical process
Data Handling and Processing Time: A major challenge, especially for large pharma consulting firms, is managing immense data volumes. Sometimes, processing and cleaning this data can take upwards of a week.
Fragmented Data Sources: Patient data comes from diverse vendors, each supplying distinct information. For example, while Electronic Health Records (EHR) provide valuable insights such as biomarker details, they’re not universally accessible. Pinpointing the appropriate Real World Data (RWD) source for specific scenarios is also challenging, and these sources are incredibly expensive.
Data Integration and Quality: Integrating data is a significant challenge, especially when vendors provide multiple tables. Even after aggregating this data, quality issues can still exist, such as missing diagnosis codes or overlooked procedures, especially if the process isn’t fully automated.
Expertise in Data Interpretation: After processing data, actionable insights must be extracted, which can be challenging due to a shortage of Subject Matter Experts (SMEs) in Patient Analytics. This scarcity complicates the interpretation of the data’s value.
Client guidance, though seldom general, prompts the need to identify and clarify objectives diligently. This crucial step provides a comprehensive understanding of tool specifics, ensuring robust data support for analytics and suitable data investments.
Innovations in Patient Analytics through AI and ML
Driven by the surge in patient data that outstrips traditional analytical tools, artificial intelligence (AI) and ML are leading the innovations in Patient Analytics. For example, chemotherapy has historically been the primary weapon against cancer but has notable side effects. Through ongoing research and innovation, targeted therapies have emerged, significantly minimizing side effects by focusing solely on cancerous cells. Alongside these advancements in treatment, diagnostic methods also progressed, detecting diseases with unprecedented precision. Yet, the growing volume of patient data presents challenges that only advanced tools in conjunction with AI and ML can effectively navigate.
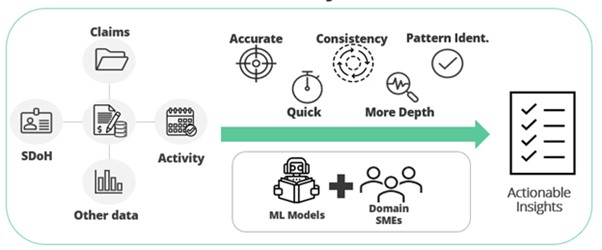
ML can uncover hidden patterns, profile patients, and predict outcomes, but it is not infallible. While it can detect trends, it may miss intricate details without expert insights. The pharmaceutical industry, replete with complex regulations and biochemistry, demands a blend of machine intelligence and human expertise. Although ML promises precision and efficiency, its ethical and accurate deployment hinges on domain specialists to guide well-informed decisions.
ML/Human Harmonization in Action
In a study involving an exceptionally effective oncology drug, ML models detected a concerning pattern: patients were discontinuing the drug around the 6-month mark. A deeper analysis found that patients with a history of renal issues were experiencing renal failure due to the drug’s intense efficacy. With this finding, pharmaceutical experts were able to intervene, integrating parallel therapies to ensure patients’ overall well-being.
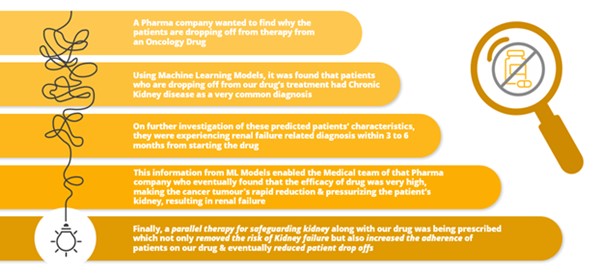
While AI and ML are undeniably transformative, their true potential is only unlocked when combined with human expertise. This ensures not only the commercial success for pharmaceutical companies but, importantly, also more effective, and safer patient outcomes.
The Future of Patient Analytics
Although historically lagging behind when it comes to tech adoption, the pharma industry is now rapidly integrating AI and ML models. These advancements promise proactive, individualized healthcare interventions, with PA at the core. A significant shift is the emphasis on Real World Evidence (RWE), which offers a comprehensive patient view and proved crucial during the COVID-19 pandemic by helping accelerate clinical trials. However, with this technological potential comes the all-too-familiar challenge of ensuring data privacy. Ethical concerns demand robust encryption, secure storage, and strict access controls. This evolution underscores a vital lesson: the balance between technological capability and ethical responsibility is paramount.
Supporting Equitable Access to Healthcare
At the heart of Patient Analytics is a profound objective: the betterment of patients’ quality of life. While this might sound like a straightforward aim, achieving it requires a delicate balance. This mission can only be accomplished if we integrate technology harmoniously with the human element.
For instance, dosage analysis has evolved from categorizing only age or gender to include ethnicity, race, and socio-economic backgrounds, emphasizing health equity. This focus on diversity is exemplified in studies of out-of-pocket expenses for specific cancer treatments, as pharma companies aim to understand if financial burdens deter patients from essential therapies. The underlying intent is clear: ensuring healthcare access is a universally equitable right, not a luxury.