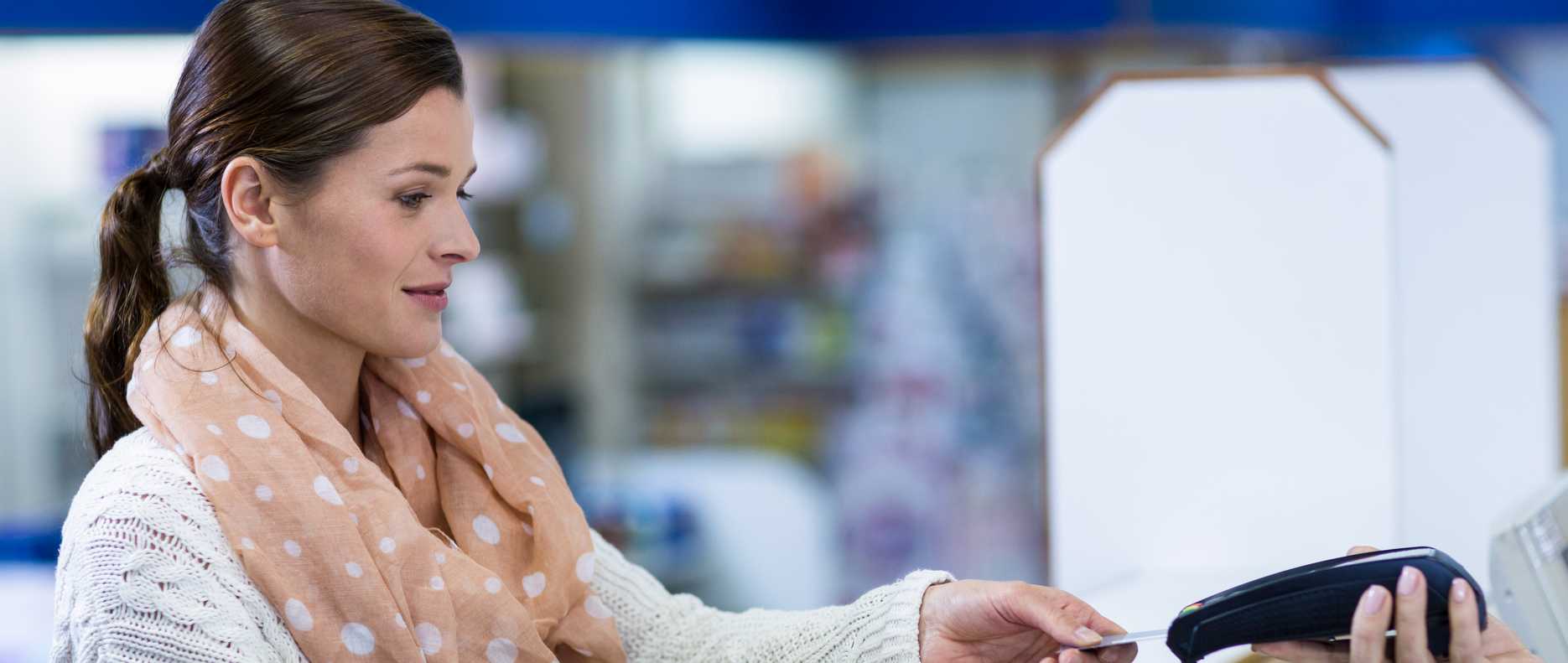
Improve collectability of the self-pay portion of medical expenses
The Big Picture
Escalating healthcare costs have forced employees with employer-provided insurance to bear a higher portion of self-payment costs such as co-pay, coinsurance, deductible and out-of-pocket expenses. Hospitals are challenged to collect the patients’ portion of medical expenses, which according to the American Hospital Association, comprises 6.1% of all services.
Multi-specialty practices collect only 56.6% of their accounts receivables in the first 30 days. Many hospitals, especially a growing number of non-profit companies, are particularly vulnerable and are seeing their access to capital weakened and their capital ratings downgraded due to bad debts.
A leading multi-specialty, multi-location healthcare provider, serving eight million patients annually, wanted to deploy analytical methods to manage and increase the collectability of the self-pay portion of services delivered.
Transformative Solution
While maximizing point-of-service collections was a key goal, improving account receivables collections post patient discharge was extremely important for hospitals. Successful risk scoring approaches for self-pay accounts required segmenting them by understanding patients’ ability as well as their willingness to pay.
To design a well-rounded collection strategy, it was critical to: know which patients required assistance with payment plans, know where to apply discounts, and define what, if any, additional collection resources were needed to be deployed.
The approach started with applying over 50 hypotheses to identify different data elements for the analysis, including patient characteristics, policy benefits, credit worthiness, and medical and procedures data to define the key drivers of patient payment behavior.
A suite of advanced predictive models was developed using machine-learning techniques to gain insights into a patient’s propensity to pay, the likely amount a patient would pay and the timeframe within which a patient was likely to pay.
Five key segments were identified with distinctive payment behaviors and the following treatments were recommended:
- Continue to bill 40% of patients with little or no additional follow-ups
- Offer payment plans and low discounts to 20% of patients to collect sooner
- Leverage collectors to target the remaining segments that were highly unlikely to pay with higher discounts.
The Change
The solution helped the provider target the right accounts, speed up collection receivables and collect more unpaid fees. The solution revealed that providers can recover significantly higher incremental dollars by selectively offering payment plans, discounts and collection resources. The client received a best-in-class solution of ensemble machine-learning models that predicted amount, time to pay and propensity to pay.
The recommendations helped the provider identify segments to collect $20MM in payments from unpaid health service invoices by targeting 30% of outstanding accounts.