From Inspection to Automation: The Role of Machine Vision in Modern Manufacturing
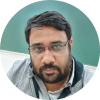
Prosenjit Banerjee
Fractal's Persona-Driven AI Chatbots: The Future of Customer Service
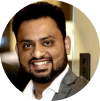
Abhijit Guha
Principal Data Scientist, AI @ Scale, Machine Vision, Conversational AI
Abhijit Guha, Principal Data Scientist at Fractal, provides a glimpse of how conversational Virtual Agents are changing the face of customer service — and beyond.
Chatbots have long been used as a customer service tool, providing clients with a DIY self- help model that can provide 24/7 assistance, answer common queries, reduce wait times, and handle multiple customers simultaneously. However, these traditional template-based models need to be improved in their functionality.
When given the option, many people still prefer dealing with human representatives, as they can provide more personalized assistance, convey empathy more effectively, and understand the complexity of nuanced challenges requiring judgment that aligns with the context of the issue. Recent advancements in artificial intelligence (AI) are about to turn this preference on its head.
Holistic customer experience with a human touch
Modern virtual agents (VA) are beyond mere chatbots. They can replicate personalities and emotional aspects of human interactions by responding to queries with personalized responses based on the user’s needs, creating a goal-oriented human-like dialogue.
The integration of these new technologies has the potential to revolutionize the way brands operate and interact with customers. Not only do virtual agent solutions allow organizations to switch to OpEx models and reduce long-term costs, but there is also massive potential for improved customer experience and satisfaction that can positively impact growth.
By deploying generative AI technology, more personalized natural language interactions between humans and machines are possible.
Many organizations are concerned about the potential of clients querying the system for information beyond the scope for which it was built. This can be dealt with by restricting the VA to a particular zone through fine-tuning, prompt engineering, and applying a moderation service to filter out inappropriate content and limit the agent’s responses to a given context. If the agent cannot answer a question from that context, it will gracefully deny the answer.
Fractal is currently working to integrate its framework with other systems where personalization is a factor. In this conversational framework, the history of the conversation is kept understanding follow-up questions better and scanning the appropriate knowledge section to provide an answer — like how the human brain works. This ability means every interaction is personalized, relevant, and seamless for each customer.
How virtual agents work
VAs work in large part because of knowledge bases. Consider how humans in an open-book examination can answer questions they don’t know the answer by referencing textbooks or notes. Their answers will depend on their access to that external knowledge. In the same way, a VA’s ability to generate precise answers to questions from a specific domain depends on the knowledge base of the VA and its capability to search for knowledge from external sources.
Fractal’s Conversational AI Framework
The creation and application of VA knowledge banks can be outlined as follows:
For example, a VA for a banking system will draw from information from a proprietary, not publicly available database and combines product and service information with personalized client data. When a user asks the VA a question, the system will search and determine the context and send that section to the system using a prompt. The VA then produces a precise or elaborated answer based on this prompt.
The goal of persona-driven VAs is to keep interaction as personalized as possible. But how can this be achieved in a world of personal and organizational personalities?
To personality or not to personality — that is the question.
The data type required for creating a personality would vary depending on the individual, organization, or department. But before that stage is reached, it’s crucial that we first understand what constitutes a personality. In its most basic form, a personality is shaped by the environment and the data gathered. This is then reflected in how it interacts with the world. Fractal’s approach converts a semantic search into a conversational agent by fine- tuning models with specific data, thereby giving VAs personalities that can be tailored to reflect the persona of the brand or organization.
However, creating a personality for an AI system is a challenging feat. Unlike humans, an AI personality is not self-regulated and requires parameters to calibrate and balance how much creativity and factual information it should contain. For example, to create an AI chatbot with Shakespeare’s personality, all the content he produced would be gathered and used as a source, allowing the chatbot to reflect his thoughts and perspectives on the world. Synthetic data can also be generated, but it may only be suitable for some scenarios: developing a personality for a banking system, for instance, would require accurate information, making synthetic data largely unsuitable.
Regardless of the data type used, privacy and data security pose the usual challenge to widespread AI creation and adoption.
Personalization vs. privacy
Developing and implementing robust security measures is the first step to addressing this concern. Organizations must ensure that their data will not leave their premises and is kept entirely under their control.
Although a VA can offer personalized service, it can only do so based on the data provided to it, and this data is filtered to ensure sensitive information is not shared. Additionally, Fractal is taking steps to prevent data leakage by exploring open-source large language models. These models could provide the exact content generation as proprietary models without compromising data.
The VA can access generic data about the bank’s products and services using our banking example. However, the centralized knowledge base does not store personalized information such as account balances and ATM pins. Instead, when a customer logs in and starts chatting, the bot will access the user’s specific information and delete any sensitive data when the conversation ends. The VA can also be deployed on a bank’s infrastructure, enhancing data security.
This approach allows for generative AI capabilities while drastically reducing the possibility of data compromise, as it ensures that each customer’s data remains within the bank’s system.
VAs beyond customer service
In the not-too-distant future, every organization could have its LLM that not only contains an extensive repository of general knowledge but is closed and smart enough to generate data within the organization’s context. However, the potential of conversational VAs extends beyond simply personalized customer service.
Fractal is developing a module called Insights and Beyond, designed to intelligently mine and interact with information in a very human-like manner. This module will be able to analyze client feedback and reviews, identifying common themes and features that answer questions about product innovation. It will also provide insights into why competitors are gaining an advantage or lagging. Organizations can use Insights and Beyond to ask their VA for factual answers and insights.
This ability can potentially revolutionize how people interact with and consume data. As the technology will require limited engineering, it will be accessible to a more comprehensive range of people. The broad impact of conversational VAs will not only influence many different industries but could also impact human life.
- Share this post