Financial decision-makers are being crushed under a deluge of data like any business function. Whether it is from ERP systems, payroll data, or ongoing financial performance data, financial decision-makers are having trouble keeping up with the influx of insights. This has resulted in them desperately looking for new methodologies and tools that can help them collect, synthesize, and act on all the data coming their way. And AI is emerging as one of the leading avenues financial teams are looking to adopt.
AI’s market value is set to explode over the next decade and will be valued at $1.3 trillion by 2030. Yet, while investment is set to pour into the space, one significant hurdle could impact technology adoption and success: transparency.
“Transparency” is a frequently bandied-about term within AI circles. However, when it comes to transparency and AI, financial operations often isn’t one of the fields that immediately jumps to mind as one of the most important. That said, it most certainly should be.
Transparency is pivotal to ensuring that financial analytics tools perform at their best – especially given the scrutiny and regulatory oversight finance functions are subjected to. The problem is that transparency is often only a secondary consideration when tools are built. The success of AI tools is either inhibited or tools are abandoned before they even get off the ground.
So with that, here are a few key steps to ensure transparency is a guiding principle in the AI-empowered financial analytics relationship. Tools can be built to catalyze the success finance functions are looking for.
Customer Involvement
No AI adoption project is easy, especially for business sectors like finance with so many stakeholders that depend on varying types of data. Financial teams, internal tech teams, and outside partners must be in lockstep regarding the needs, goals, and prospective outcomes. In addition, there needs to be a clear understanding and alignment on how a tool needs to function to meet each business arm’s different expectations and preferences. For example, while some stakeholders like frontline analysts may prefer tools that give them the “nitty-gritty” details needed to make advanced decisions, this granularity maybe unwieldy and unnecessary for top-level decision-makers who just need a more scaled-back picture of overall business performance. Therefore, staking out the framework and functionality early by engaging with each vested interest can engender buy-in and ensure a transparent rapport is established even before a tool is put into production.
Appoint Change Managers
Change can be difficult and stressful, and when delays occur, downright discouraging. It can be so discouraging that businesses can even pull the plug on projects that could be pivotal in boosting and modernizing their business. To prevent this from happening and help projects navigate choppy waters, financial departments and their partners both need to appoint change champions and advocates. They can help keep projects moving and maintain morale on both sides of the relationship when a delay or stumbling block occurs. Perhaps more importantly, these change champions can help keep partners honest and flag concerns so that lines of communication remain open, transparency around progress and functionality stay intact, and no surprises occur once a tool is launched.
Build with security in mind
One of the first questions that any financial decision-maker is going to ask is, “how secure is our data?” And rightfully so, given the amount of personally identifiable data financial teams are privy to. Thus, perhaps more so than when it comes to any other element of the tool they are building, AI companies need to build a complete glass box around what security measures are in place and the ongoing maintenance and improvement of these measures. In addition, AI service providers need to construct tools that make it very easy to wall off data on a user-by-user basis so that only the parties that need specific data can access it. Moreover, tools need to be put in place to track which users have tried unsuccessfully to access specific data to monitor potential internal threat actors.
—
The best way to build transparency within finance departments regarding AI adoption and use is to ensure that every aspect of the process from the relationship to production to ongoing maintenance is as clear and open as possible. If not, financial teams will continue to struggle to unlock the broad potential of AI and make the best decisions possible.
Authors
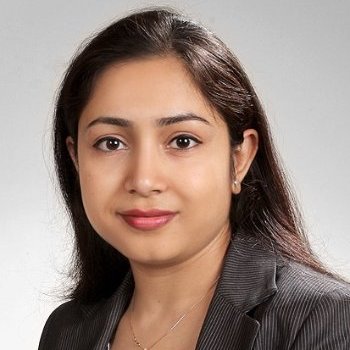