Data platforms represent a pivotal change from traditional storage and database systems. These integrated technology suites streamline organizational data to enhance decision-making, allowing businesses to focus on customer experience, revenue growth, and innovation.
Cloud providers offer all-in-one solutions that simplify infrastructure management plus come with a suite of a variety of Data and AI services to address enterprise business needs. The advent of Generative AI (GenAI) is accelerating this transformation. GenAI grants instant access to innovations and facilitates rapid prototyping and market entry.
Current landscape
For many organizations, data platforms serve as crucial organizational infrastructure, enabled by cloud technology to offer scalable and agile data management solutions. These platforms make it easy to manage different data types, whether structured, semi-structured, or unstructured. They can handle tasks that need to be done all at once or in real time, and their centralization makes it easy for engineers and data scientists to use self-service analytics. This helps them create and deploy data models and products quickly and effectively. Flexibility means organizations can quickly change to meet new business needs, keeping a solid base regardless of how complex the data or demand becomes.
Approach & Building blocks of success
Data platforms are typically built using a bottom-up approach, where the focus is on bringing the source data in its original form onto the public cloud or hybrid cloud through an agile and iterative process based on business priorities.
However, the right data stack will significantly vary for a large enterprise vs a startup in any domain primarily due to factors like organizational scale and complexity, budget, resource availability, infrastructure, legacy systems and integration, compliance and regulatory needs, governance, and business priorities.
A data platform for a large enterprise or a startup should at least have these five essential features and functions that are crucial for success. Each of these features is supported by essential functions that meet the evolving needs of organizations:
Feature | Description |
---|---|
Accessibility | Depending on their permissions, people in the organization can access the data they need, improving collaboration and decision-making. |
Flexibility | It integrates seamlessly with external and on-premises systems, creating a unified ecosystem for data and allowing data to move easily. |
Scalability | Expands to handle more data as needed, ensuring that different business tasks can be done efficiently. |
Security | Implements robust measures to protect sensitive information, enforcing stringent protocols to maintain data integrity and compliance with regulations. |
Automation | Streamlines data pipeline creation and transformation, making operations more efficient and allowing businesses to adjust quickly. |
Function | Description |
---|---|
Storage and processing layer | Supports data storage, retrieval, and processing, acting as the platform’s backbone. |
Data ingestion, transformation, and orchestration layer | Ensures data is accurately captured, transformed, and orchestrated across systems for optimal use. |
Governance layer | Establishes policies for accessing data, ensuring its quality and compliance. This encourages responsible and ethical use of data. |
Consumption layer | Enables end-user access and analysis of data, supporting informed organizational decision-making. |
Observability feature | Provides insights into the platform’s health and performance, ensuring issues are promptly addressed. |
These core features and functions comprise the modern data platform, which helps businesses work better, develop new ideas, and stay competitive.
Evolving approaches
The strategic shift toward viewing data as a product marks the breakaway from traditional data management approaches. It requires both technical evolution and a cultural shift within organizations.
Organizations that have relied on monolithic data systems often encounter substantial challenges. The centralization of data management, executed by specialized teams overseeing various data sources, necessitates domain-specific expertise. Consequently, significant bottlenecks emerged, particularly in the realms of data ownership, quality, and prioritization, complicating the management of fluctuating data needs and volumes.
The data mesh architecture offers enhancements over traditional monolithic systems by conceptualizing data as a distinct product, effectively addressing ownership, quality, and scalability issues. Each team is responsible for its own data in this framework, leading to heightened data quality and relevance. This model advocates for distributed systems and shared governance, which aligns the data strategy with organizational objectives and empowers teams with control over their data assets. By pinpointing the needs of data consumers and fostering standardization and collaboration, the data mesh paradigm amplifies flexibility and cooperation across the data ecosystem.
Fractal helps finance, retail, consumer goods, and healthcare organizations build and improve modern data platforms and customize solutions to fit specific industry needs . At the heart of our innovation are Quark and Certifi, tools designed to refine data platform operations.
Quark
Quark facilitates the creation of efficient data pipelines and semantic products without being tied to any specific cloud platform. Its user-friendly interface allows those familiar with cloud services to rapidly transform data and create semantic layers, speeding up development by up to 50%.
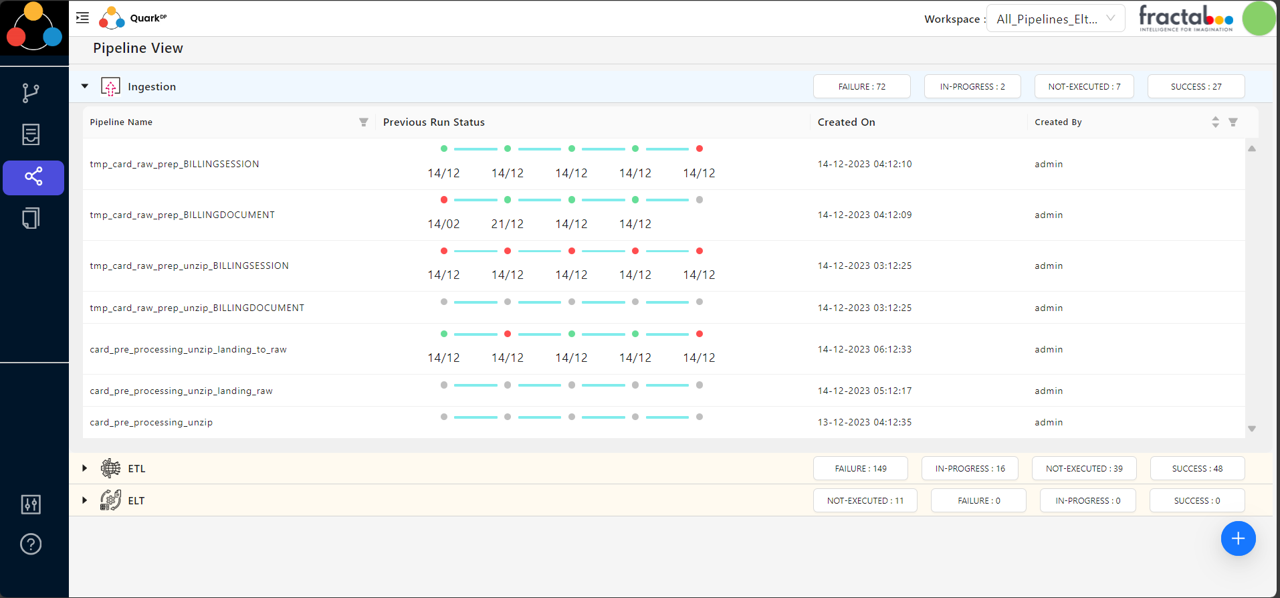
Quark’s versatility is underscored by its support for over 50 operators and 25 connectors. It’s compatible with primary cloud services like GCP, Azure, AWS, Snowflake, and Databricks. This tool exemplifies Fractal’s commitment to enhancing data platforms’ agility and responsiveness.
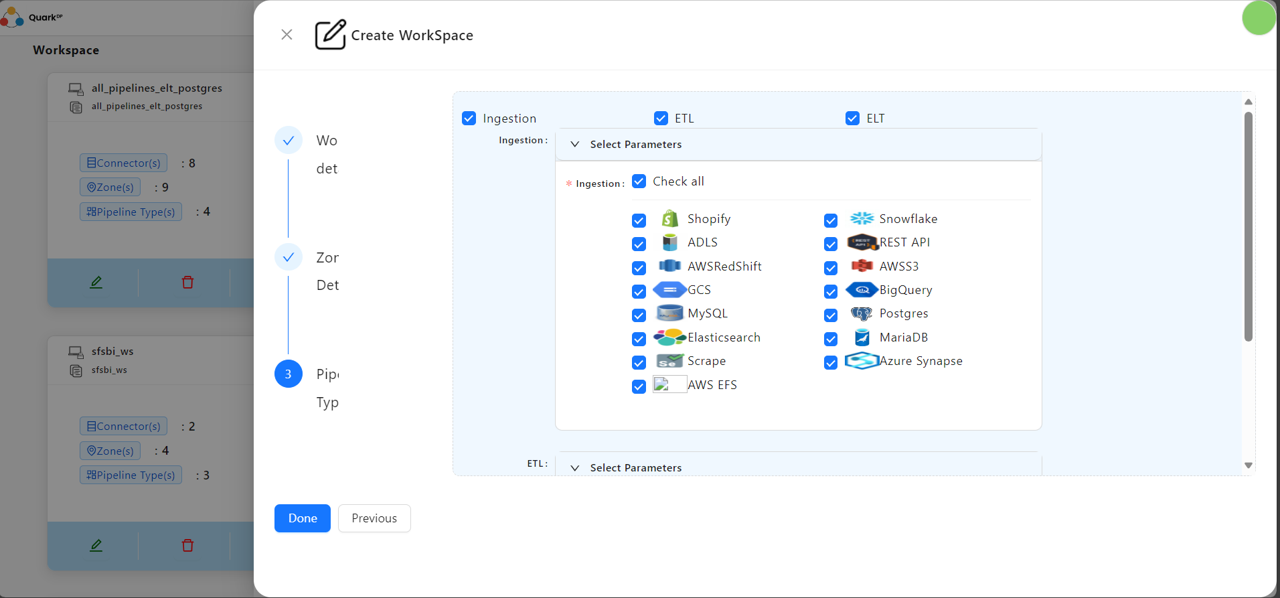
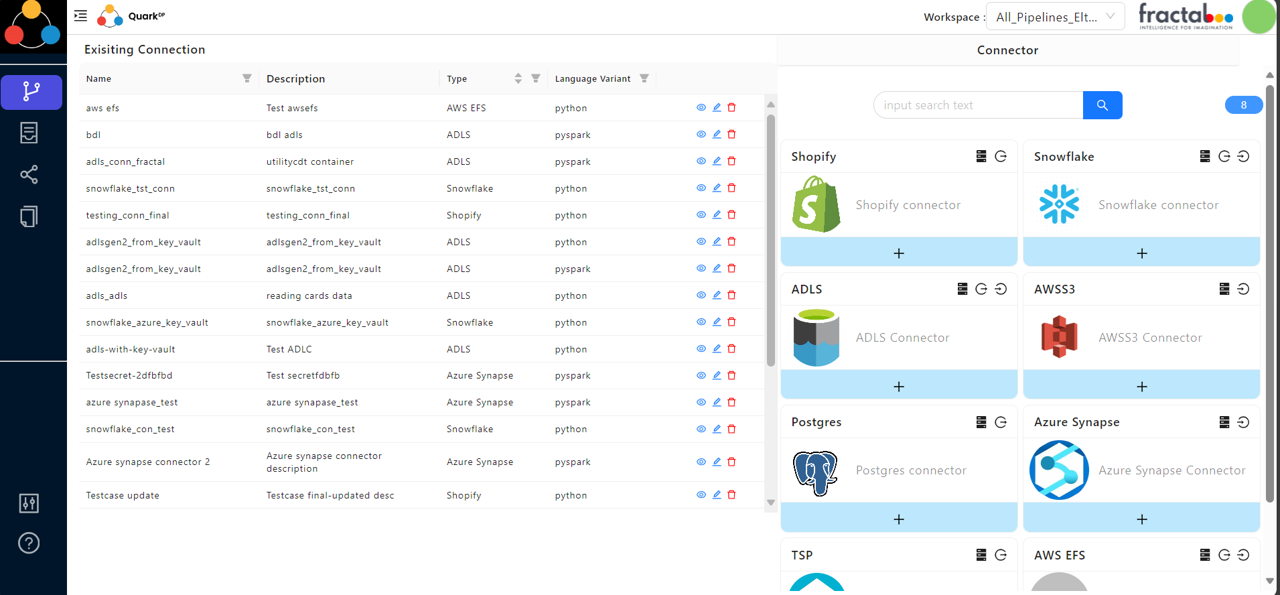
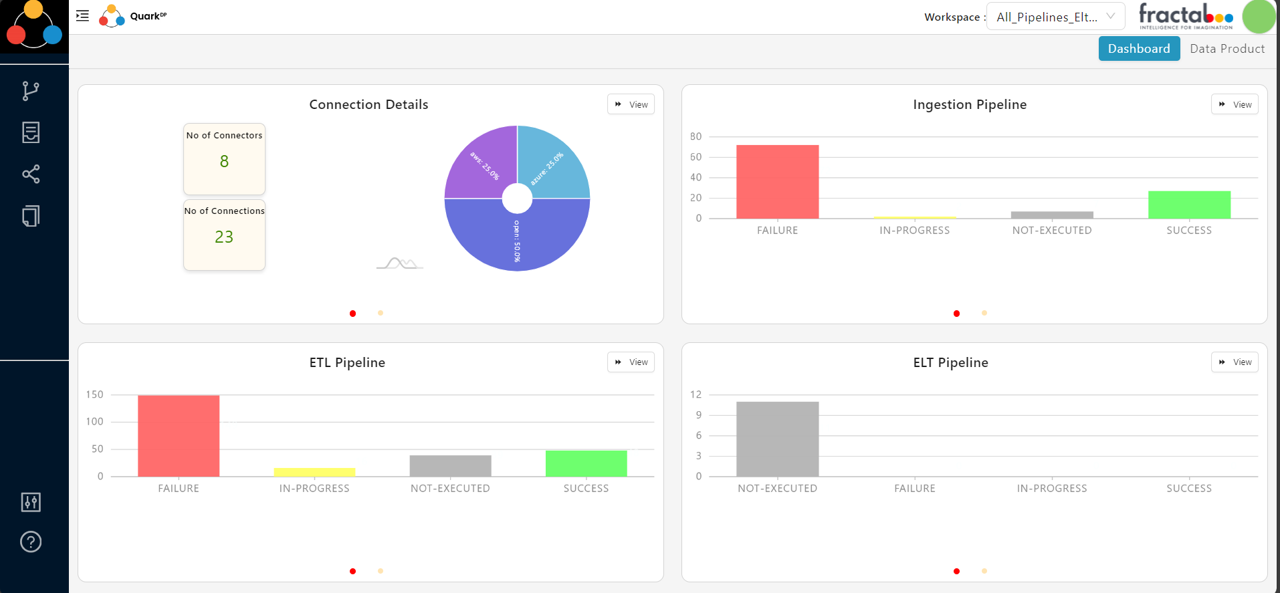
Certifi
Certifi is a cloud-agnostic data quality engine that ensures consistent and accurate data across platforms. It comes with predefined rules for organizing columns and checking the data structure, and it can also handle custom rules for specific organizational needs.
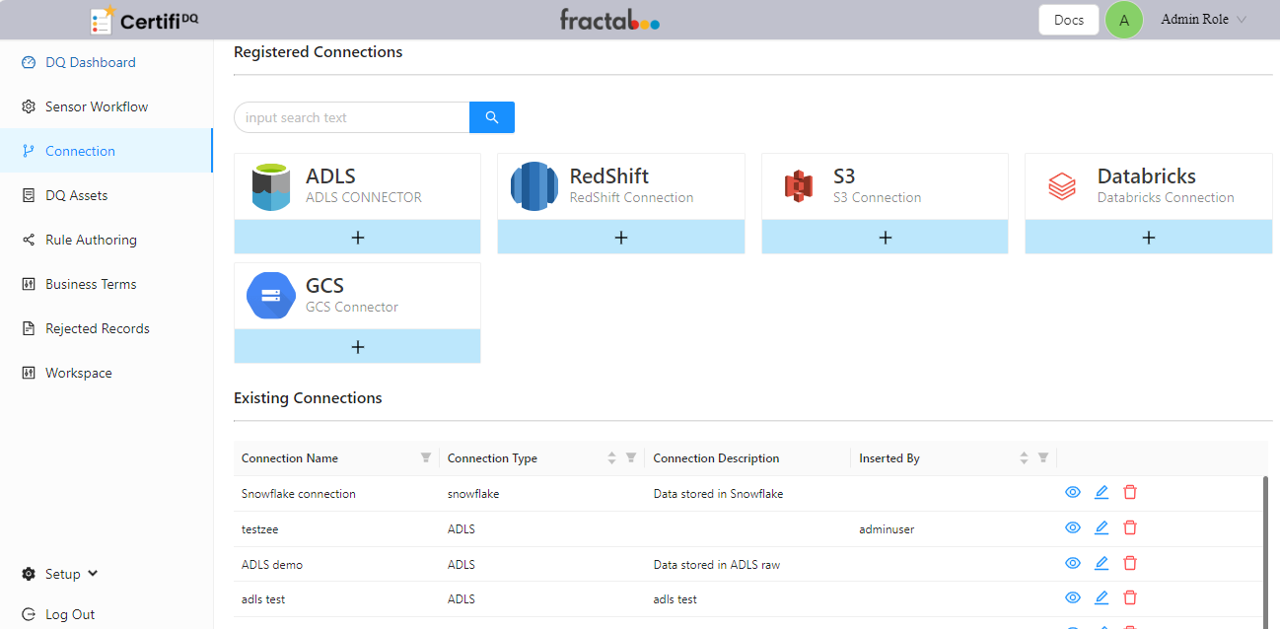
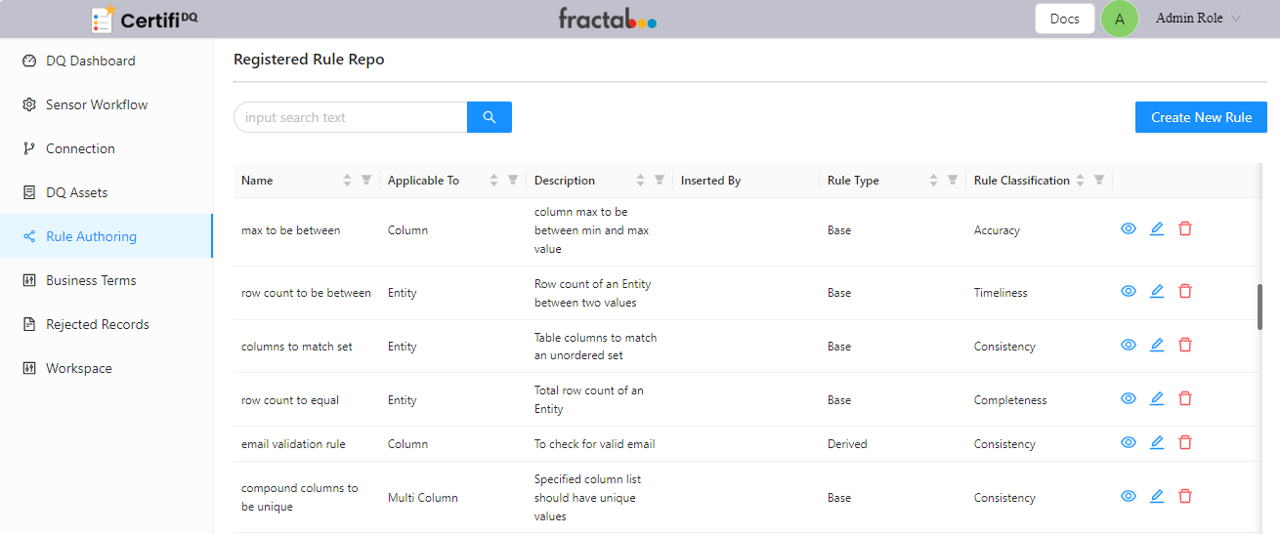
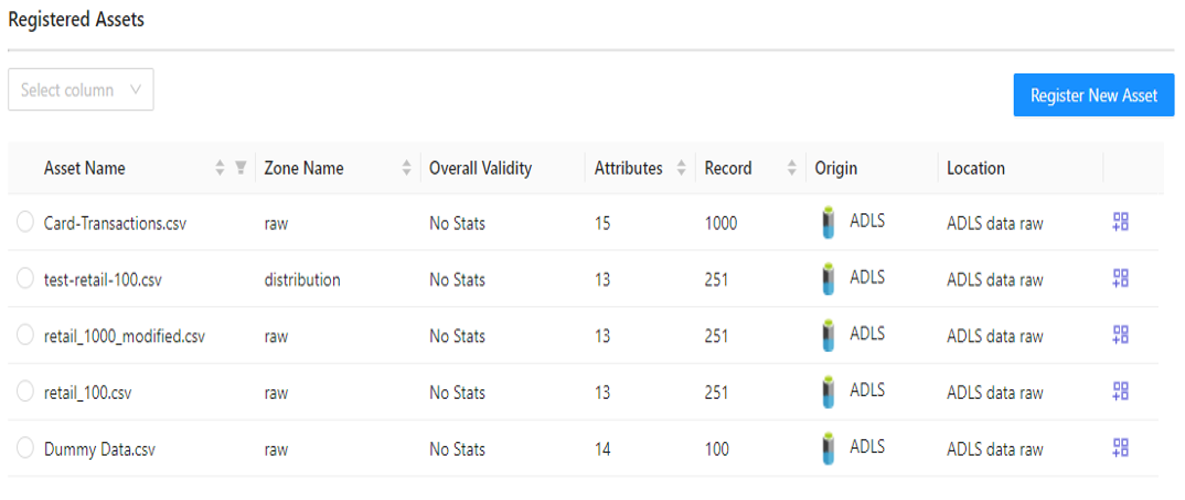
This capability is crucial for preserving high data quality before further development, mitigating potential issues in the future. Certifi’s effectiveness has been demonstrated across various client sectors, particularly in consumer goods, where it aids in swiftly adopting new quality standards.
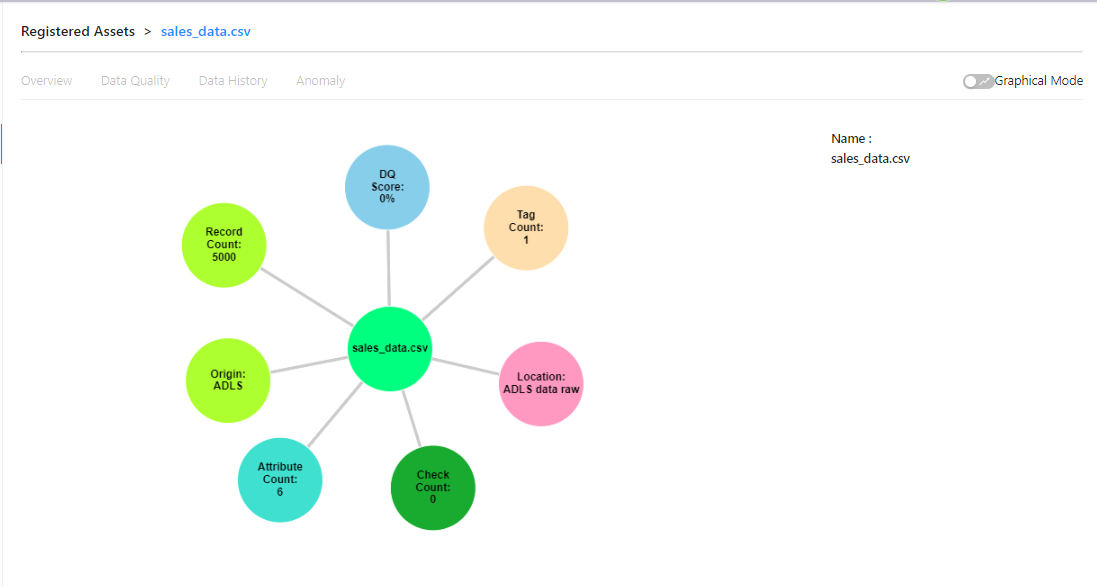
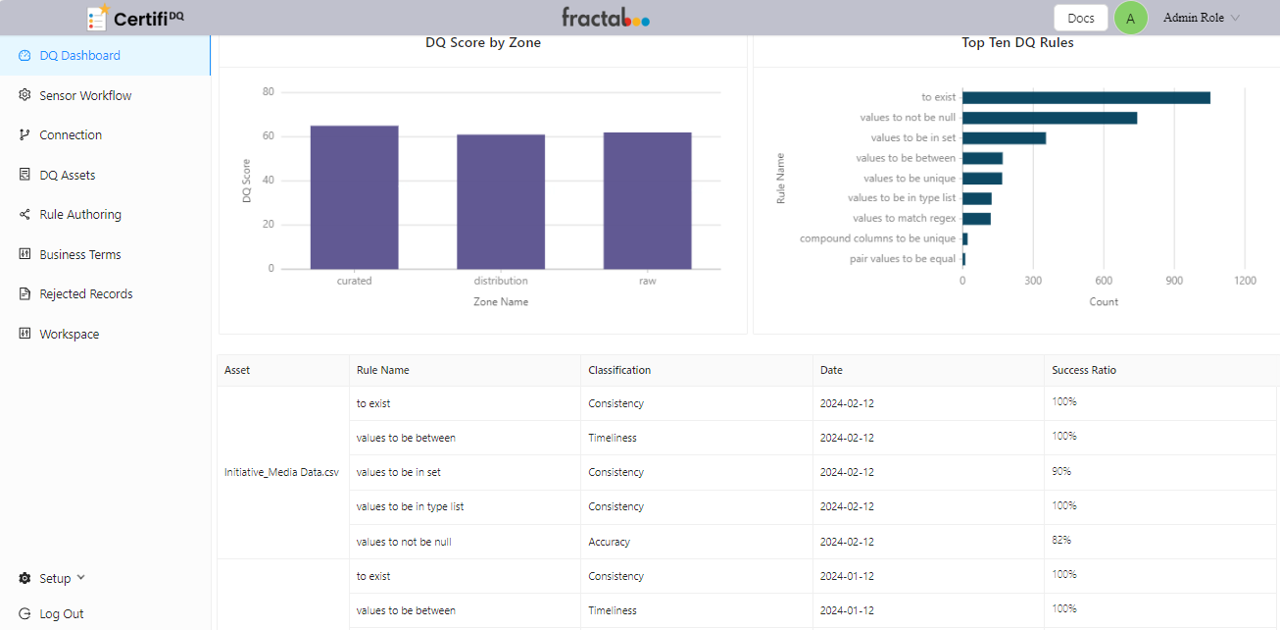
Our suite of tools expedites the development of data platforms, ensuring alignment between technical capabilities and strategic objectives.
Challenges and solutions
However, adopting data mesh and product thinking has its challenges. It means reassessing an organization’s capabilities, undergoing a cultural shift, developing talent, and potentially collaborating with partners. The journey requires thorough readiness assessment and commitment to cultural and technical adaptations.
Looking ahead
The data platform landscape is poised for transformative change, with GenAI leading the charge. This improvement makes data management quicker, smarter, and more flexible, meeting the growing demand for timely and accurate business insights. For instance, it can automate moving from old systems like Teradata to Google Cloud’s BigQuery, saving time and effort by converting scripts instantly to fit BigQuery.
The integration of GenAI into data platforms reflects a broader shift towards automation, streamlining the adoption of new technologies and enhancing platform functionality. To remain competitive amid these changes, organizations must embrace these technologies, fostering a culture of innovation and adaptability. Investing in GenAI and similar advancements enables data platforms to meet business objectives efficiently.
Organizations must embrace emerging technologies like GenAI to thrive and expand. This means changing the culture to focus on continual learning and innovation. A close examination of the organization’s current capabilities would be needed. This includes fostering a culture that embraces change and recognizes the evolving importance of data, particularly as businesses transition from traditional to modern data systems. Collaborative efforts and experimentation with GenAI are integral to leveraging its full potential in tasks such as data organization, comprehension, and utilization in machine learning, ultimately enhancing operational efficiency and extracting valuable insights.
Training employees to learn new skills is essential for quickly creating and using the latest solutions. This helps teams handle present and future challenges. Keeping up with what’s happening in the industry helps organizations proactively predict changes and develop new ideas. This ensures they remain competitive and responsive to the changing needs of customers.