While large language models (LLMs) have captured headlines and sparked immense interest, their full transformative potential lies in the next phase – embedding AI agents directly into enterprise workflows. The post-LLM era has arrived, and businesses must prepare for agentic AI to alter how work gets done.
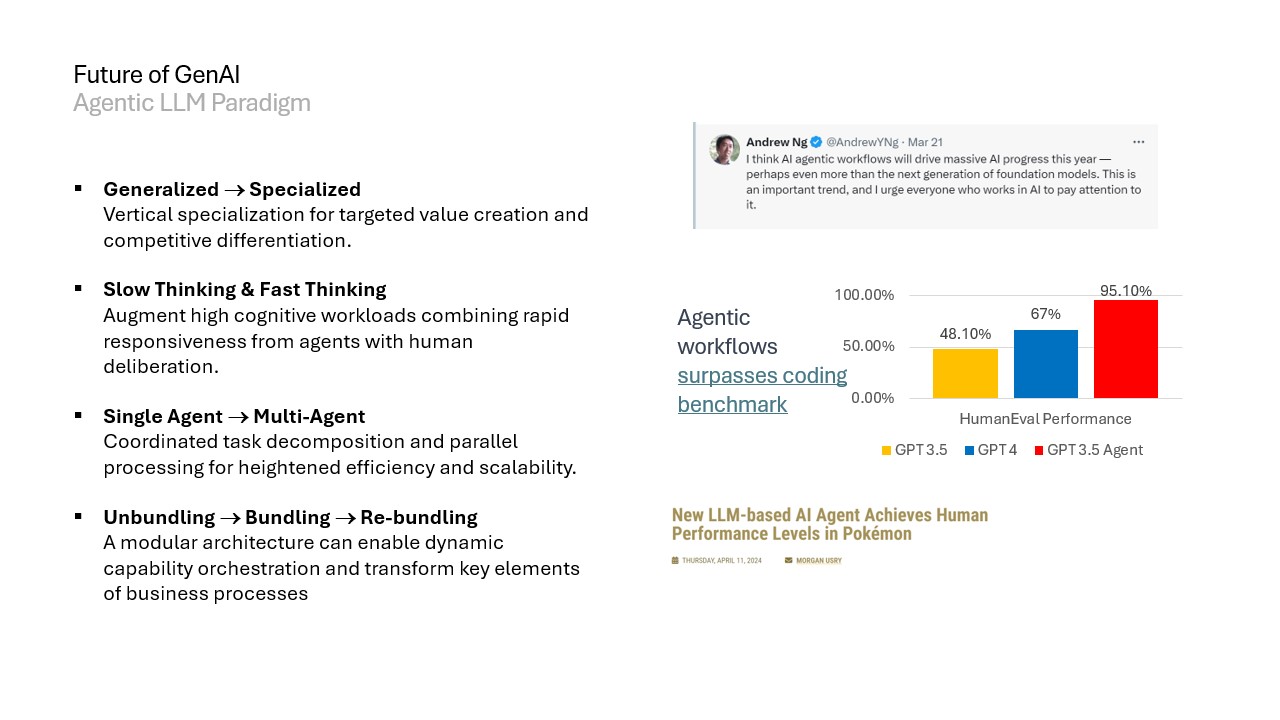
What are Agentic AI Workflows?
Agentic AI workflows involve integrating advanced AI language models, which I’ll refer to as AI agents, into an organization’s core business processes and operations. These AI agents can understand complex queries, analyze data, formulate solutions, and initiate actions while collaborating seamlessly with human teams.
LLM Agents are the future of enterprise-wide adoption of Gen AI. They harness core LLMs and integrate them with existing workflows to optimize processes and enable meaningful decisions.
Why is it relevant now?
The rapid advancement of large language AI has opened the floodgates for realistic, responsive, and multi-talented AI assistants. Yet, their actual value lies in their operational integration versus utilizing LLMs as isolated tools. Several converging factors make agentic AI workflows particularly compelling now:
1.Technology readiness: While basic language models have existed for years, recent breakthroughs in areas like multi-modal learning, constitutional AI, and predictive modeling have elevated AI agents to new levels of capability. They can now ingest and correlate information from multiple sources, understand complex contexts, formulate original insights, and even initiate actions based on constraints – bringing us closer to general artificial intelligence.
2. Business opportunity: The global economy is emerging from disruptions, cropping up vulnerabilities in human-centric processes and workforces. Companies seek opportunities to enhance resilience, agility, and productivity through increased automation. AI agents provide a path to streamline workflows and reduce manual labor dependencies in both back-office operations and customer-facing processes.
3. Human and consumer acceptance: Despite initial fears, society has grown accustomed to AI assistants through ubiquitous experiences like chatbots and digital assistants. This familiarity, combined with publicized examples of AI’s potential upsides, has heightened trust among businesses and individuals to embrace AI’s expanding role in higher-value tasks – setting the stage for the adoption of embedded agentic AI workflows.
4. Market forces: The competitive advantages realized by early adopters of agentic workflows could create an “AI workflow gap” that accelerates further adoption. Enterprises may have little choice but to invest in embedding AI agents to keep pace with their AI-augmented competitors’ efficiency/throughput improvements. This cyclical dynamic foreshadows aggressive investment and innovation in this space.
With rapidly maturing AI capabilities combined with prevailing business needs and societal openness, the stage is set for enterprises to seriously explore and operationalize agentic AI workflows. Those who move decisively can secure decisive competitive advantages.
For the enterprise:
From supply chain optimization to customer service process flows, nearly every business function can potentially benefit from agentic AI workflow transformation. AI agents can serve as intelligent advisory partners, accelerate analysis, automate tasks and documentation, and elevate decision-making quality—all on demand within existing systems.
What about Robotic Process Automation (RPA) vs agentic AI workflows
While agentic AI workflows represent the next transformative frontier, it’s essential to differentiate them from prior automation approaches like robotic process automation (RPA). Both can play critical roles, but understand the key distinctions:
RPA strengths
RPA has been valuable for automating high-volume, repetitive tasks that follow defined rules and structured data inputs/outputs. It excels at replicating routine, tedious actions across systems/applications to boost throughput and reduce human labor needs. Common RPA use cases include data entry, transaction processing, report generation, and system integration.
RPA limitations
However, RPA operates strictly based on its programmed instructions and structured dataflows. It cannot handle exceptions, understand context, or exercise judgment for more complex, unstructured processes involving human decisions and inputs. Scaling RPA across an enterprise also requires extensive process mapping and coding for each workflow.
Agentic AI strengths
Agentic AI workflows bring increased intelligence to enable advanced automation and human-AI collaboration. Rather than simply following coded rules, AI agents can adapt to contextual nuances, understand and respond to open-ended inputs, formulate insights, and initiate actions based on constraints—just as a skilled human employee could.
This allows AI agents to augment human teams for more complex, unpredictable processes like customer service, content creation, analysis, and decision support across domains. Their ability to seamlessly interact with humans while flexibly handling ambiguity drives immense efficiency and quality gains.
Complementary deployment
RPA and agentic AI are not an “either/or” proposition. The highest value will come from thoughtfully implementing both:
• Leverage RPA for commodity automation of repetitive, high-volume tasks.
• Layer agentic AI on top of standardized RPA workflows to handle exceptions.
• Deploy AI agents for complex, judgment-intensive processes unsuitable for RPA.
Embarking on this dual-track approach positions enterprises to optimize their process landscape and realize the full compounding benefits of intelligent automation across structured and unstructured work domains.
Process Characteristic | RPA Suitability | Agentic AI Workflow Suitability |
---|---|---|
The process is rules-based with structured inputs/outputs. | High | Low |
The high volume of repetitive tasks | High | Low |
The process requires handling exceptions or ambiguity | Low | High |
The process requires contextual understanding and judgment | Low | High |
Need for human-AI collaboration on the same process | Low | High |
Processes span multiple apps/systems in complex ways | Medium | High |
We have deeply assessed where Agent workflows are better suited vs. RPA.
Where to begin:
While compelling, integrating AI agents is not simple. It requires a strategic roadmap spanning process mapping, systems integration, governance frameworks, and workforce reskilling. Enterprises should start by pinpointing the highest-impact opportunities, defining desired outcomes, understanding existing gaps, and planning proof of concept.
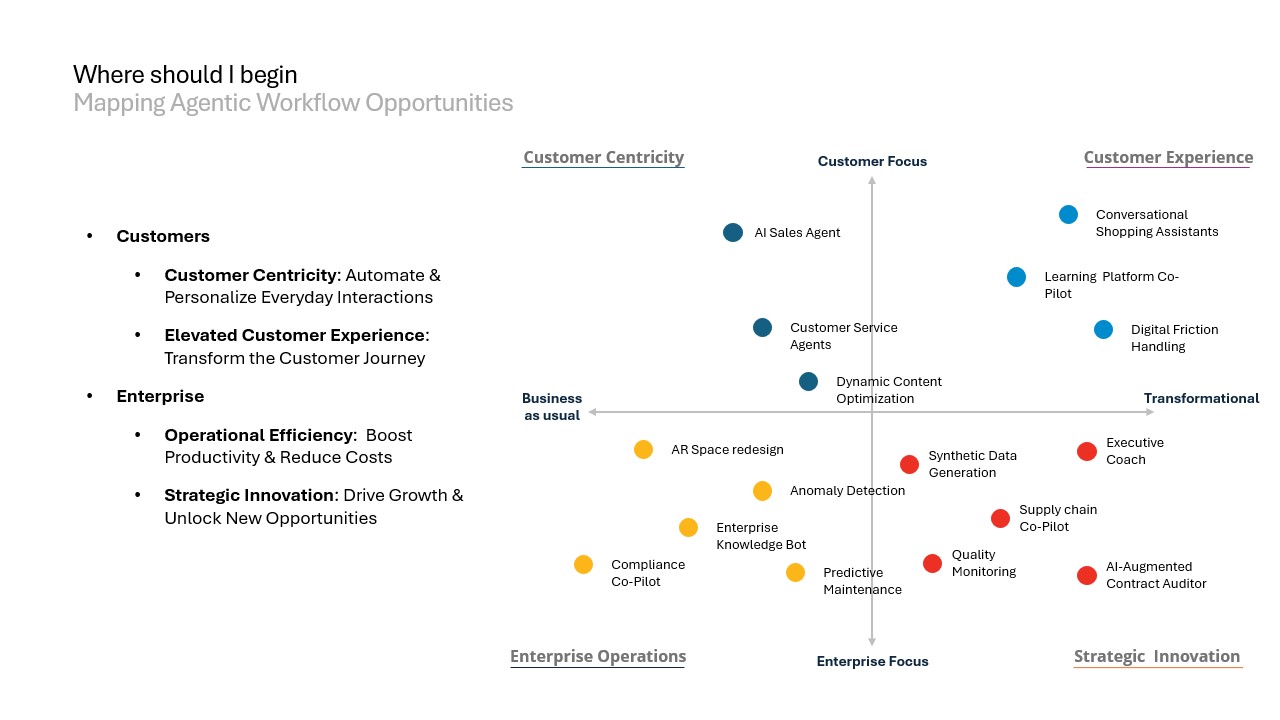
Begin with strategic opportunities and functional transformation
Conduct an enterprise-wide process inventory paired with capability assessments of AI agents. This allows pinpointing processes that are great candidates for embedded AI due to:
• High human labor costs or constraints
• Excessive handoffs, delays, and friction points
• Needs for real-time intelligence and decision support
• Opportunities to fundamentally re-imagine processes
Prioritize processes core to strategic objectives like productivity acceleration, customer experience enhancement, cost optimization, and operational resilience. Cross-map to AI agents’ current and projected capabilities to build out use case roadmaps.
Accelerate productivity | Enhance customer experience | Transform enterprise operations |
---|---|---|
Every enterprise has workflows ripe for productivity breakthroughs through intelligent automation. Key areas include:
• Employee/team augmentation for research, analysis, documentation • Decision support systems with on-demand data synthesis • Streamlining internal processes like HR, finance, legal • Turbo-charging high-value work like product/service innovation |
Embedding agentic AI directly into customer-facing processes allows transforming experiences through heightened personalization, immediacy, and cognitive intelligence, such as:
• Omni-channel, personalized conversation agents • Guided digital self-service tools and advisory • Intelligent case management and conflict resolution • Data-driven, dynamic experience optimization |
AI agents can fundamentally reshape operations and elevate performance by interfacing across systems, functions, and partners to optimize workflows like:
Embedding agentic AI directly into customer-facing processes allows transforming experiences through heightened personalization, immediacy, and cognitive intelligence, such as:
• Intelligent supply chain monitoring and intervention • Dynamic pricing, marketing, and channel orchestration • Predictive maintenance and mitigation systems • Risk monitoring and automated controls/compliance |
The path forward starts by mapping these strategic priorities to existing workflows and capabilities. First, pursue succinct, high-ROI deployments to build capabilities while developing a holistic, long-range vision for enterprise-wide augmentation.

The LLM agent decision framework
Integrating agentic AI workflows: A progressive approach to enterprise automation The Decision Framework is designed to guide organizations through the progressive journey of their today and tomorrow, starting from basic Robotic Process Automation (RPA) and advancing to fully autonomous AI operations. This framework helps decision-makers understand the various stages of AI integration, identify where their organization currently stands, and plan a strategic roadmap for future AI adoption.
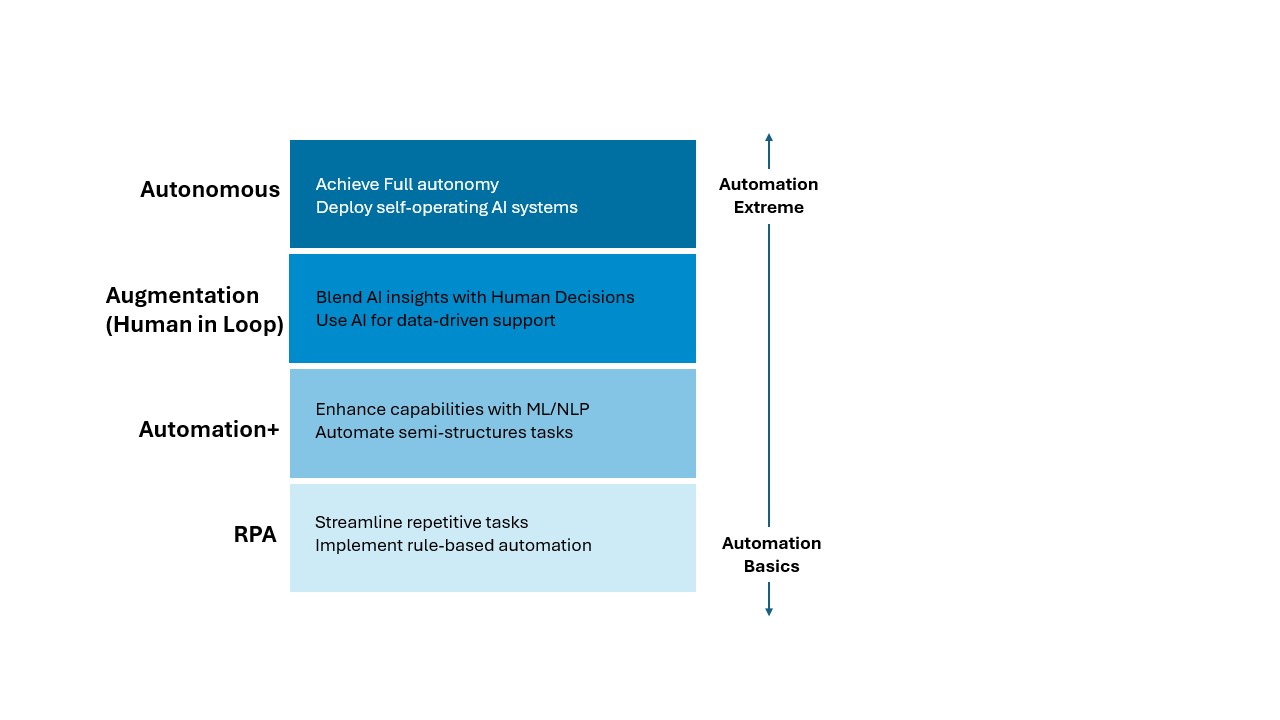
The table below summarizes the key characteristics and objectives for each stage in the automation journey. It provides a snapshot of what each stage entails and the strategic value it brings to the organization.
RPA Laying the foundation | Automation+ efficiency | Augmentation Human-machine synergy | Autonomous Achieving full automation |
---|---|---|---|
Streamline repetitive, rule-based tasks. | Incorporates machine learning and natural language processing | Combines AI insights with human decision-making | AI systems operate independently with minimal oversight. |
Essential for initial efficiency improvements | Enhances service delivery and operational insights | Optimizes human-AI collaboration for superior outcomes | Drives operational excellence and competitive advantage |
Before diving into advanced AI solutions, organizations must assess their current state, define clear objectives, identify opportunities, and develop a detailed roadmap. A concise guide for enterprises to follow, ensuring a strategic and effective implementation of AI technologies.
1. Current state assessment | 2. Define objectives | 3. Identify opportunities | 4. Develop a roadmap |
---|---|---|---|
Determine where the organization currently operates on the matrix. This could be at the “RPA” stage (Robotic Process Automation), “Automation+”, “Augmentation (with Human in Loop,”),” or “Autonomous” | Establish clear goals for what level of automation is to be achieved. These could range from basic RPA to full autonomy. | Look for processes that can be automated or augmented with technology. This could involve identifying repetitive tasks that can be automated or areas where AI can enhance human decision-making | Create a step-by-step plan moving from RPA towards augmentation and eventually autonomous operations. This roadmap should consider the organization’s current capabilities, resources, and business objectives. |
The era of AI-driven transformation is upon us. While Large Language Models (LLMs) have demonstrated AI’s immense potential, the real game-changer for enterprises lies in embedded AI agents that directly enhance human capabilities. Organizations strategically embracing this shift will gain sustainable competitive edges through smarter, more automated, and highly efficient operations. The time is ripe for business leaders to explore and implement agentic AI workflows, positioning their companies at the forefront of this technological revolution.
Reference –
Agentic Design Patterns Part 1 Four AI agent strategies that improve GPT-4 and GPT-3.5 performance. (2024, March 20). DeepLearning.AI. https://www.deeplearning.ai/the-batch/how-agents-can-improve-llm-performance/