Summary
Our client, one of the UK’s largest P&C insurance companies, offers consumers and businesses a wide range of insurance products, including motor, home, business, travel, and more. They faced challenges in their call center operations, with long hold times and errors affecting efficiency.
To address this, they partnered with Fractal to implement FractalGPT – GenAI Knowledge Assist. This AI-driven solution improved knowledge retrieval and response generation, leading to smoother operations and better customer interactions.
Challenge
The insurer faced challenges due to long hold times in its contact center, stemming from the difficulties faced by the agents in locating relevant information related to policies and procedures.
This resulted in response delays, frequent supervisor interventions, and inaccurate responses.
- A large workforce of over 500 agents managing high volume of calls exceeding 300,000 per month.
- Approximately 30% of calls requiring escalation to supervisors.
- About 10% of interactions resulting in errors leading to potential financial damages.
Solution
To solve these problems, the insurer partnered with Fractal, a leading provider of B2B AI solutions.
Fractal implemented its FractalGPT – GenAI Knowledge Assist application. FractalGPT is an AI-powered solution designed to revolutionize knowledge retrieval within large enterprises using the power of large language models and generative AI.
In eight weeks, FractalGPT- GenAI Knowledge Assist was deployed and customized on the client’s AWS environment, where it was integrated with the internal SharePoint and policy repositories and several other backend tools. The virtual assistant was further customized by training it on thousands of documents covering various topics such as policy FAQs, claims, premium payment, policy closure and more.
It leveraged a large language model (LLM) to perform semantic search and response generation, ensuring that the answers were relevant and consistent with the source documents. The approach ensured that no confidential or company-specific information left the company tenant.
Knowledge Assist, built on Amazon EKS, Amazon Bedrock, and Amazon Open Search Service, ensures efficient and accurate information retrieval without extensive training. It seamlessly handles complex documents, including those containing tables, across multiple file formats such as PDFs, Docs, and PPTs. Additionally, the solution integrates with OCR to extract information from images.
Result
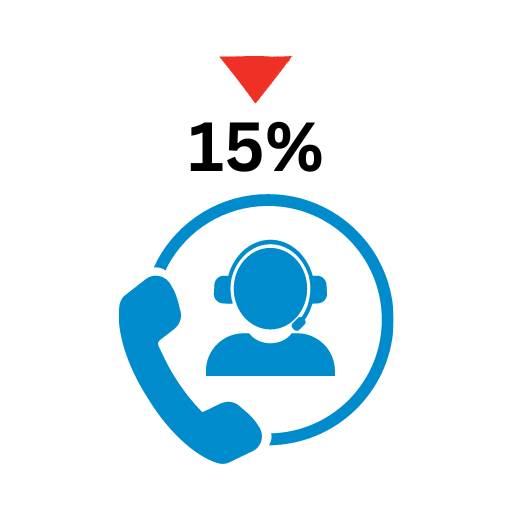
Reduction in AHT
Streamlined interactions, faster resolutions
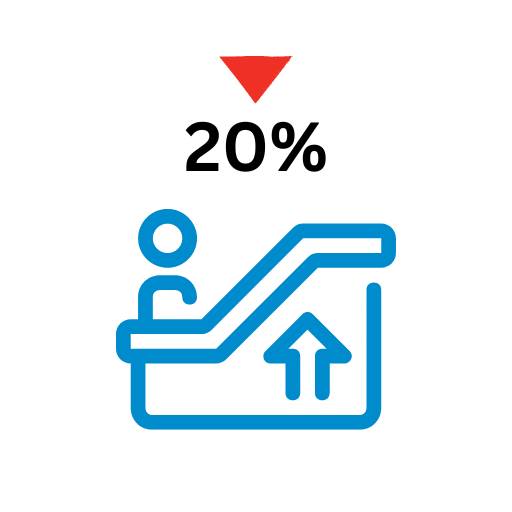
Reduced supervisor escalations
Agents empowered to handle queries independently
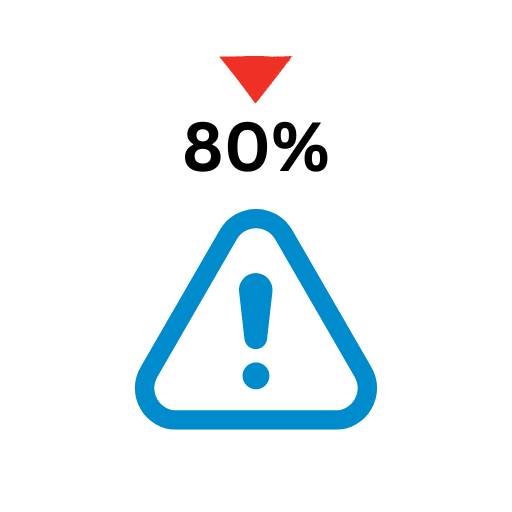
Decrease in erroneous response
Accuracy soared
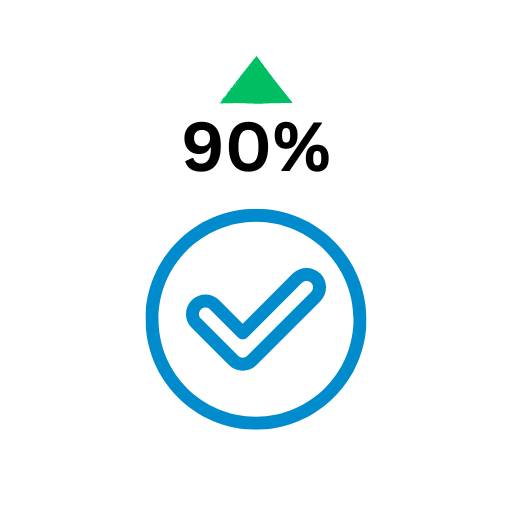
Answer accuracy
Boosted customer satisfaction
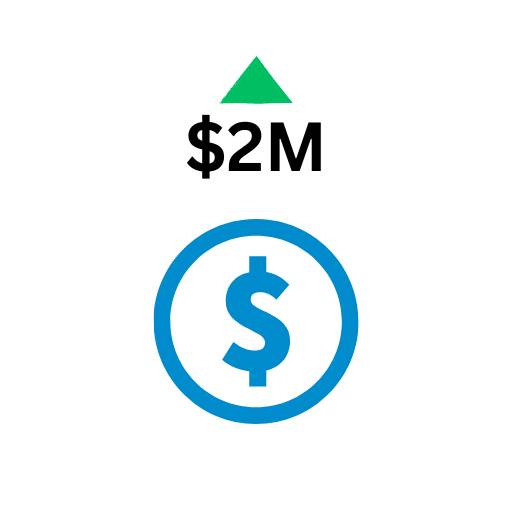
Projected annual savings
A substantial impact on the bottom line
FractalGPT solution is now available on the AWS Marketplace; click here to learn more.