The retail industry is one of the most dynamic and fast-paced sectors, comprised of various stakeholders engaged in selling finished products to end-user consumers. In 2022, the U.S. retail sector was estimated at more than seven trillion dollars. The sector is projected to continue to grow, and by 2026 U.S. retail sales are expected to reach approximately USD 7.9 trillion. With the increased demand for consumer goods in different sectors and the ever-increasing choices of products at low costs, investments in the retail sector have also grown over the past few years.
As there is always an element of change in this industry, new challenges are encountered daily. Take product stockouts, for example. Suppose a customer walks into a grocery store to purchase items of their favorite brand but discovers the product is unavailable. Frustrated by this, the customer chooses to either buy another brand or postpone the purchase; both scenarios are unfavorable to the business. The brand image and sales of the product are damaged because of this out-of-stock issue. The out-of-stock situation occurs when the inventory of a particular product is exhausted; this causes a problem for suppliers and retailers.
Multiple reasons could cause the product stockout, such as inaccurate inventory data, lack of demand forecasting, or an unseasonal spike in purchasing. Many of these underlying causes of stockouts can be avoided if the business implements adequate processes to be carried out every month.
To avoid situations like the stockout example above, retail companies need to develop a methodology for streamlining the following operations:
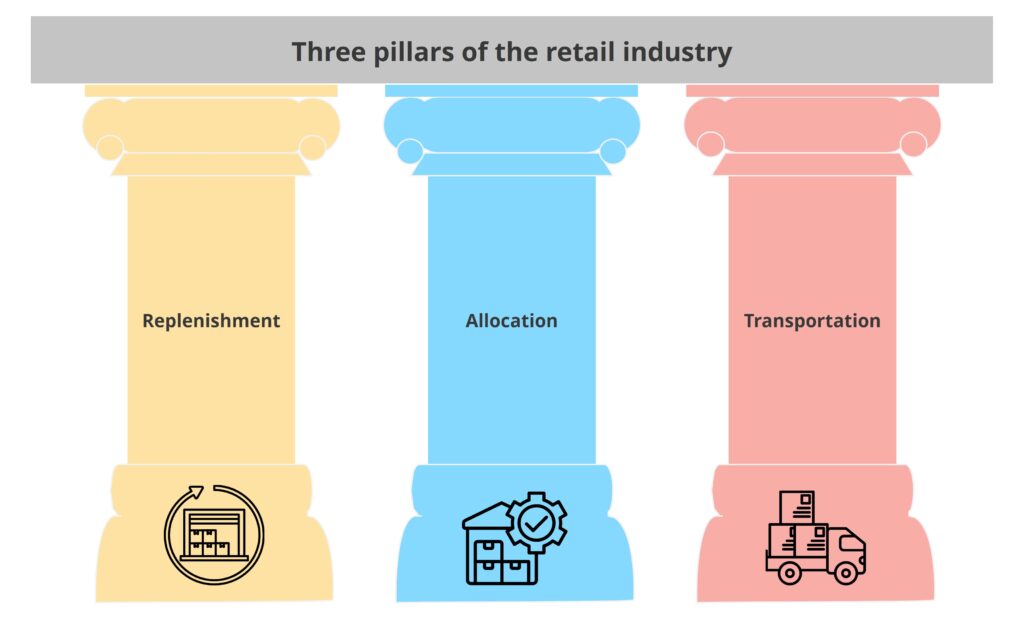
- Replenishment
- Allocation
- Transportation
These three operations create the three pillars of the retail industry that help monitor real-time insight into customer behavior and understand their buying patterns, hence strengthening the retail foundation.
1. Replenishment
Replenishment refers to a situation where the amount of stock left in the store is counted so that the right products are available in an optimal quantity. It is considered an essential aspect of inventory management as it ensures that the right products are being reordered to meet the customer demand.
In operational terms, the efficiency of store replenishment has a significant impact on profitability. The effectiveness and accuracy of store ordering affect sales through shelf availability and storage, handling, and wastage costs in stores and other parts of the supply chain. By optimizing demand forecasting, inventory management, and setting order cycles and order quantities by making them more systematic, the gains obtained are significant, often amounting to savings of several percent of total turnover.
For companies that must manage many SKUs, one of the most effective ways of making store replenishment more accurate, efficient, and cost-effective is by using a replenishment system specifically tailored to business operations. When many different products need to be managed, manual ordering is highly labor-intensive and expensive; this results in companies using the replenishment system.
An efficient replenishment system reduces process costs, improves inventory turnover, and provides higher service levels. The system constantly monitors the stock, sales, and demand while considering the forecast changes in demand and adjusting the replenishment orders. The company can control its inventory to ensure long-term profitability by recognizing the sales frequency, value, or profit margin. The replenishment system calculates the safety stock level for each SKU separately and sets them to meet the service level targets with efficiency, considering the predictability of demand.
2. Allocation
In allocation, the new stock of products is distributed to individual store units to maximize the product’s sales and prevent any stock-out situation in the future. This process enables assigning supplies to support the organization’s strategic goals. Having sufficient stock levels is an essential component for any retail business; with the changing consumer habits, it becomes crucial for the stock to be available in the right place at the right time.
To meet new and increasing demands, retailers need an efficient process to gather, interpret, and analyze data from customer behaviors and habits, which would help get a more localized and specific idea of what is sold at a larger quantity in different locations. Items that are high sellers in one particular area may not sell well in others, so recognizing and monitoring this can ensure that the stock is allocated to the most needed location. Due to this, an opportunity is provided for the retailers to encourage sales by pushing stock of a similar type that a customer may favor at a particular location.
3. Transportation
Transportation plays a significant role in delivering the right stock of products at the right point of delivery. It connects the business to its supply chain partners and influences customer satisfaction with the organization. With the ever-changing customer preferences and as their expectations continue to evolve, the transportation industry is undergoing a dramatic transformation to meet these demands.
Today, data plays a vital role in shaping the industry’s progress amidst tough competition. Due to the maturation of automation technologies, AI will help the transportation industry to manage drivers and fleet managers. By employing the techniques of AI, fleet and truck adjustments will offer data in real-time, eventually improving the industry’s standard. The safety and retention of the drivers will also increase from these newly acquired standards, and with enhanced access to data, there will be transparent communication between drivers and carriers.
The average time between goods purchasing and delivery decreases by using real-time findings, making retailers focus on transportation to improve their business performance. The ability to automate insights, alerts, and data exchange more quickly will be the game-changer for this industry.
These three pillars of retail can be strengthened by adopting in-house solutions and capabilities like StockView for retail, Edge video analytics, and Dynamics 365 customer insights.
How could these solutions help the retail industry?
StockView for retail helps retailers reduce lost sales and improve customer experience by automatically detecting out of stock items on shelves. It uses computer vision technology running at the edge to detect gaps on store shelves automatically. It also provides retailers with powerful insights and analytics into stock-out activities at both single-store and multi-store levels.
Powered by Microsoft Azure Stack Edge, it offers a scalable, flexible, and cost-effective solution that brings the power of the Azure cloud platform down to the individual store, eliminating the need for costly and unreliable data transfers while offering a predictable and consistent TCO (Total Cost of Ownership).
Edge Video Analytics solutions for retail typically leverage Azure Stack Edge devices, IoT devices, vision AI (Artificial Intelligence), and other technologies in conjunction with in-store cameras or other video sources.
Insights gained from Edge Video Analytics can allow retailers to swiftly react to in-store customer behavior and product stock-outs while improving security and reducing shrinkage.
Also, organizations can track store foot traffic, automatically notify employees to open more checkouts due to lengthy queues, and automatically detect product stock-outs. These insights can be used to help improve demand forecasting, optimize supply chains, and detect variances in population preferences down to the individual store.
Dynamics 365 customer insights give you access to Microsoft’s extensive library of pre-built data connectors to help businesses gain faster time to value. Plus, you can power up your customer profiles with AI by leveraging Azure Machine Learning and Azure Cognitive Services, and Fractal’s library of custom AI models. So, we leverage this solution to maximize the potential of customer data.
All these solutions and capabilities help understand the customer motivations, preferences, and desires to meet their demands and increase sales effectively, strengthening the retail industry’s pillars.
Conclusion
To meet these growing customer expectations, retailers should prioritize collecting customer data and analyzing it to support business decisions throughout their value chain. The inventory stocking patterns and shipping routes will shift in relation to patterns informed by this data. Retailers should make a concentrated effort to leverage the data while making critical business decisions, and to remain efficient; they must remain flexible and transform their operations as they capture more insights from their data.
Over the past 20+ years, Fractal has helped many retail companies make their replenishment, allocation, and transportation operations more efficient by leveraging AI, engineering, and design. If you would like to learn more about optimizing these aspects of your retail business, please contact us to speak with one of our experts.
Find how Fractal helped a global CPG company to operationalize an analytics engine designed and provide store recommendations for maximizing investments in their Azure environment. Read the full case study here: https://fractal.ai/casestudies/store-level-insights/